Welcome!
The QUARRG Lab is located at Queen’s University in Kingston, Canada. We conduct research on autonomous robotics, including quadcopters, ground vehicles, and robotic arms. The laboratory is equipped with world-class equipment, providing our researchers with the opportunity to develop advanced projects involving multiple artificial intelligence disciplines.
Ensuring the safety and reliability of both ground and aerial autonomous vehicles is a critical aspect of our research.
— Dr. Sidney Givigi
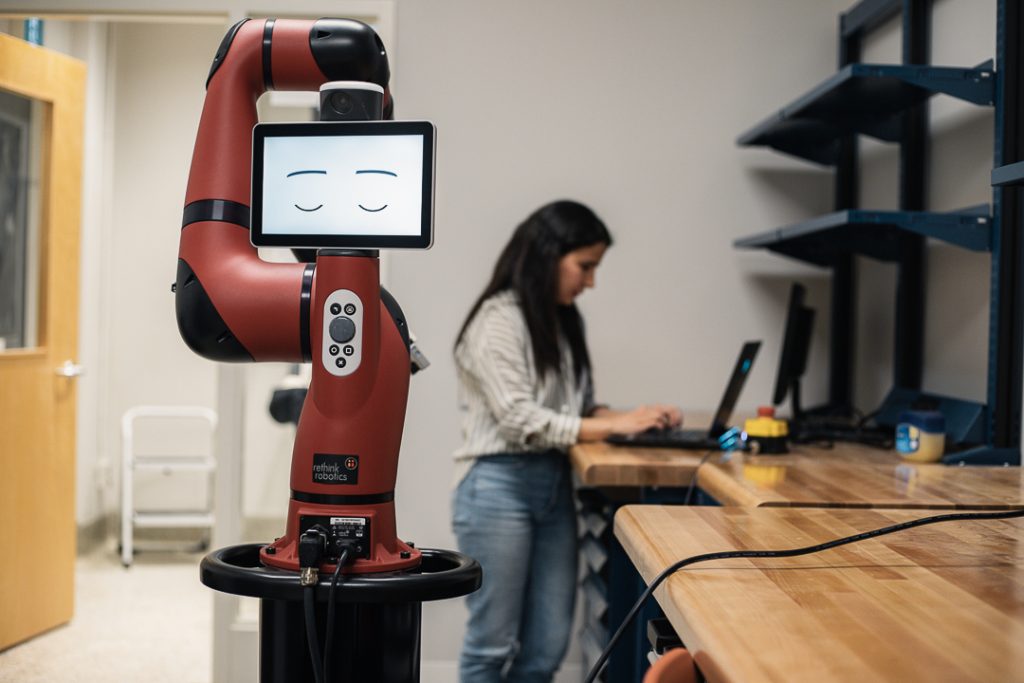
Our Research
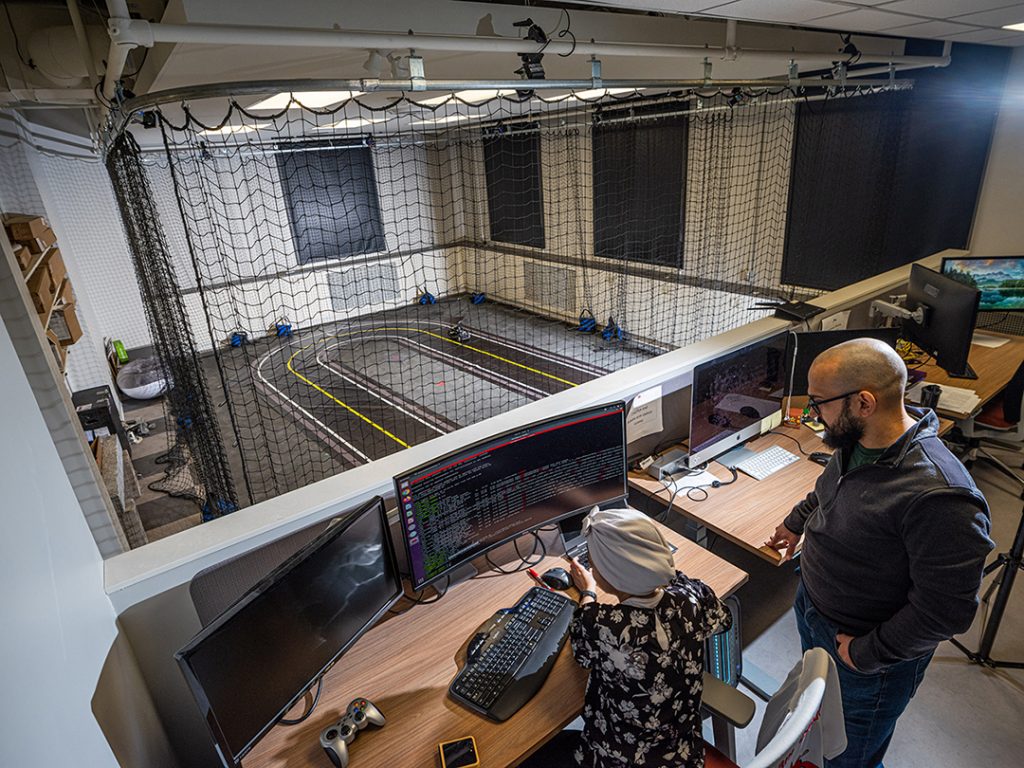
Autonomous Vehicles
The QUARRG research on autonomous vehicles using machine learning (ML) is at the cutting edge of transforming transportation. ML is pivotal in enabling vehicles to perceive their environment, make informed decisions, and navigate complex scenarios with minimal human intervention, especially when integrated with traditional or classical techniques.
At QUARRG, researchers focus on perception for both aerial and ground vehicles within the context of smart transportation systems. They utilize data from sensors such as cameras, LIDAR, radar, and GNSS to train deep learning models that enhance the vehicles’ ability to localize, recognize crucial objects and landmarks, and interpret environmental conditions in real time. These ML models empower vehicles to perceive and understand their surroundings in a manner akin to human drivers. The team at QUARRG is also advancing the efficiency of learning methods by incorporating knowledge from the physics and engineering principles underlying vehicle navigation.
By leveraging mathematical modeling, we design models to be robust and capable of generalizing across diverse, dynamic conditions and varying weather. This requires rigorous testing and validation, both in simulation and through real-world experiments, to prepare autonomous vehicles for the myriad scenarios they might encounter.
Machine Learning
QUARRG has worked extensively in the design of new machine learning algorithms that combine different mathematical formulations. Among them, we have proposed algorithms that use causal inference to speed up learning. We have also combined knowledge of the structure of a problem with reinforcement learning (RL) to improve the performance of algorithms. This has resulted in hybrid algorithms that perform better than traditional methods. These algorithms are ported to ground vehicles and drones that operate independently of each other. Our algorithms have also been ported to robotic arms to assist children with neuromotor disabilities to interact with their environments.
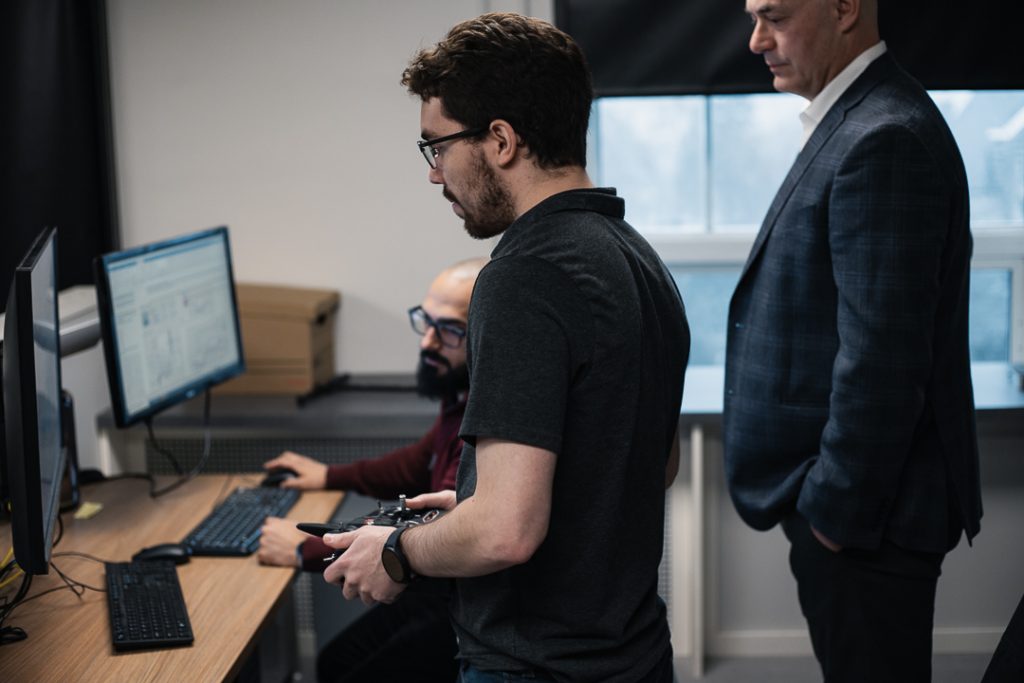
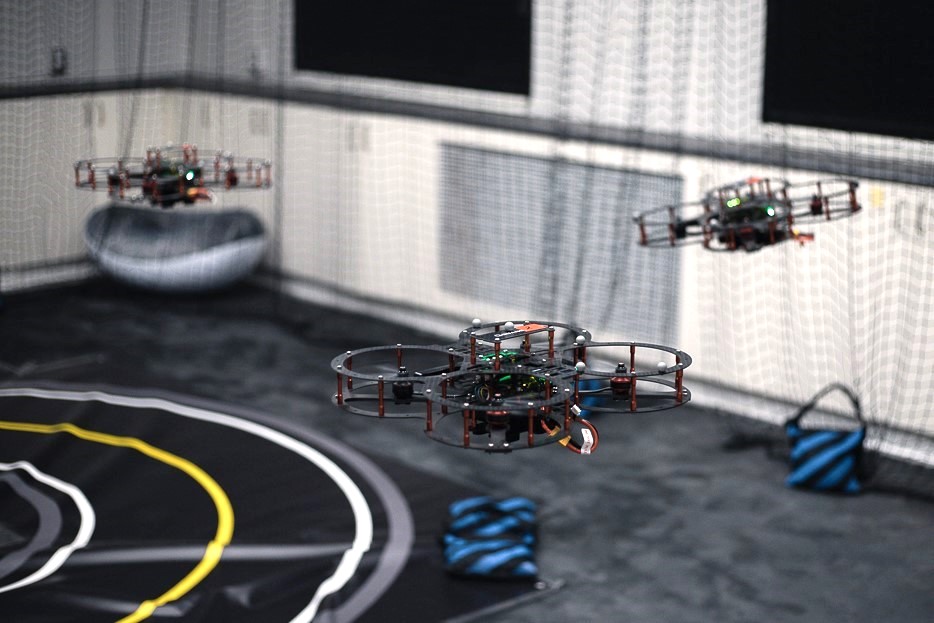
Robotics
We design advanced solutions for robotic systems by integrating classical methods with cutting-edge machine learning approaches. Much like humans, robots need to plan the tasks they must perform. At QUARRG, we have explored various planning methods, seamlessly integrating them with controllers to enhance vehicle guidance, environmental mapping, and spatial coverage.
One of our areas of focus is developing algorithms that enable vehicles to make split-second decisions in dynamic environments. By using reinforcement learning (RL) coupled with classical control techniques, such as Model Predictive Control (MPC), vehicles learn optimal control strategies through trial and error, improving their ability to handle unexpected situations like sudden obstacles or erratic behavior from other vehicles.
Our researchers also focus on how multiple vehicles can collaborate to perform complex tasks while using limited communication. Several different methods for task and motion planning have been pioneered by our researchers. Using our techniques, groups containing several agents have been able to solve difficult multi-agent problems such as the assembly of structures, coordinated coverage of regions, and monitoring of infrastructure.
Contact Us
Email: quarrg@queensu.ca
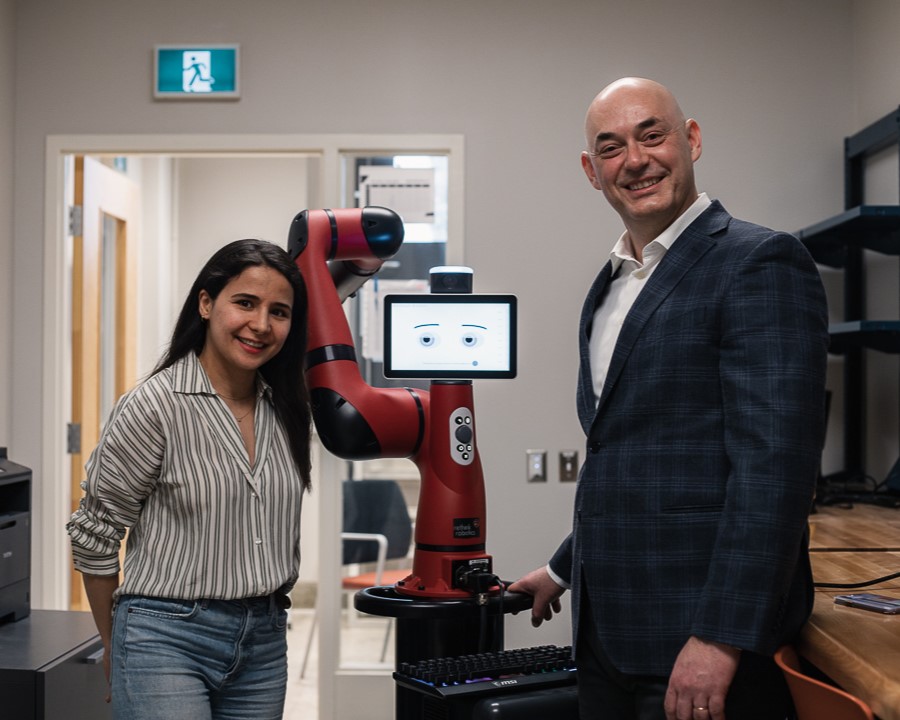