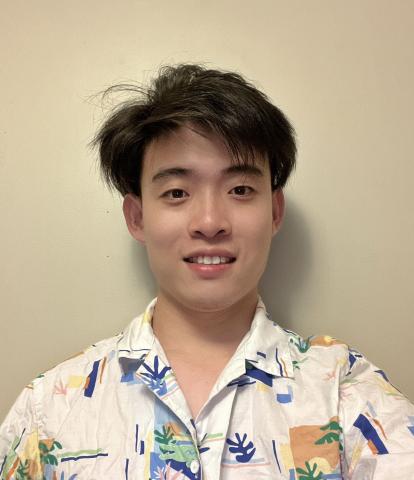
Yang, Jianming; Hisey, Rebecca; Bierbrier, Joshua; Law, Christine; Fichtinger, Gabor; Holden, Matthew
Frame Selection Methods to Streamline Surgical Video Annotation for Tool Detection Tasks Journal Article
In: pp. 892-898, 2024.
@article{yang2024,
title = {Frame Selection Methods to Streamline Surgical Video Annotation for Tool Detection Tasks},
author = {Jianming Yang and Rebecca Hisey and Joshua Bierbrier and Christine Law and Gabor Fichtinger and Matthew Holden},
year = {2024},
date = {2024-01-01},
pages = {892-898},
publisher = {IEEE},
abstract = {Given the growing volume of surgical data and the increasing demand for annotation, there is a pressing need to streamline the annotation process for surgical videos. Previously, annotation tools for object detection tasks have greatly evolved, reducing time expense and enhancing ease. There are also many initial frame selection approaches for Artificial Intelligence (AI) assisted annotation tasks to further reduce human effort. However, these methods have rarely been implemented and reported in the context of surgical datasets, especially in cataract surgery datasets. The identification of initial frames to annotate before the use of any tools or algorithms determines annotation efficiency. Therefore, in this paper, we chose to prioritize the development of a method for selecting initial frames to facilitate the subsequent automated annotation process. We propose a customized initial frames selection method based on …},
keywords = {},
pubstate = {published},
tppubtype = {article}
}
Given the growing volume of surgical data and the increasing demand for annotation, there is a pressing need to streamline the annotation process for surgical videos. Previously, annotation tools for object detection tasks have greatly evolved, reducing time expense and enhancing ease. There are also many initial frame selection approaches for Artificial Intelligence (AI) assisted annotation tasks to further reduce human effort. However, these methods have rarely been implemented and reported in the context of surgical datasets, especially in cataract surgery datasets. The identification of initial frames to annotate before the use of any tools or algorithms determines annotation efficiency. Therefore, in this paper, we chose to prioritize the development of a method for selecting initial frames to facilitate the subsequent automated annotation process. We propose a customized initial frames selection method based on …
Yang, Jianming; Hisey, Rebecca; Bierbrier, Joshua; Fichtinger, Gabor; Law, Christine; Holden, Matthew
Frame Selection Methods to Streamline Surgical Video Annotation for Tool Detection Tasks Conference Forthcoming
IEEE, Forthcoming.
@conference{nokey,
title = {Frame Selection Methods to Streamline Surgical Video Annotation for Tool Detection Tasks},
author = {Jianming Yang and Rebecca Hisey and Joshua Bierbrier and Gabor Fichtinger and Christine Law and Matthew Holden},
publisher = {IEEE},
abstract = {Given the growing volume of surgical data and the
increasing demand for annotation, there is a pressing need to
streamline the annotation process for surgical videos. Previously,
annotation tools for object detection tasks have greatly evolved,
reducing time expense and enhancing ease. There are also many
initial frame selection approaches for Artificial Intelligence
(AI) assisted annotation tasks to further reduce human effort.
However, these methods have rarely been implemented and
reported in the context of surgical datasets, especially in cataract
surgery datasets. The identification of initial frames to annotate
before the use of any tools or algorithms determines annotation
efficiency. Therefore, in this paper, we chose to prioritize the
development of a method for selecting initial frames to facilitate
the subsequent automated annotation process. We propose a
customized initial frames selection method based on feature
clustering and compare it to commonly used temporal selection
methods. In each method, initial frames from cataract surgery
videos are selected to train a surgical tool detection model.
The model assists in the automated annotation process by
predicting bounding boxes for the surgery video objects in the
remaining frames. Evaluations of these methods are based on
how many edits users need to perform when annotating the
initial frames and how many edits users are expected to perform
to correct all predictions. Additionally, the total annotation cost
for each method is compared. Results indicate that on average,
the proposed cluster-based approach requires the fewest total
edits and exhibits the lowest total annotation cost compared
to conventional methods. These findings highlight a promising
direction for developing a complete application, featuring
streamlined AI-assisted annotation processes for surgical tool
detection tasks.},
keywords = {},
pubstate = {forthcoming},
tppubtype = {conference}
}
Given the growing volume of surgical data and the
increasing demand for annotation, there is a pressing need to
streamline the annotation process for surgical videos. Previously,
annotation tools for object detection tasks have greatly evolved,
reducing time expense and enhancing ease. There are also many
initial frame selection approaches for Artificial Intelligence
(AI) assisted annotation tasks to further reduce human effort.
However, these methods have rarely been implemented and
reported in the context of surgical datasets, especially in cataract
surgery datasets. The identification of initial frames to annotate
before the use of any tools or algorithms determines annotation
efficiency. Therefore, in this paper, we chose to prioritize the
development of a method for selecting initial frames to facilitate
the subsequent automated annotation process. We propose a
customized initial frames selection method based on feature
clustering and compare it to commonly used temporal selection
methods. In each method, initial frames from cataract surgery
videos are selected to train a surgical tool detection model.
The model assists in the automated annotation process by
predicting bounding boxes for the surgery video objects in the
remaining frames. Evaluations of these methods are based on
how many edits users need to perform when annotating the
initial frames and how many edits users are expected to perform
to correct all predictions. Additionally, the total annotation cost
for each method is compared. Results indicate that on average,
the proposed cluster-based approach requires the fewest total
edits and exhibits the lowest total annotation cost compared
to conventional methods. These findings highlight a promising
direction for developing a complete application, featuring
streamlined AI-assisted annotation processes for surgical tool
detection tasks.
increasing demand for annotation, there is a pressing need to
streamline the annotation process for surgical videos. Previously,
annotation tools for object detection tasks have greatly evolved,
reducing time expense and enhancing ease. There are also many
initial frame selection approaches for Artificial Intelligence
(AI) assisted annotation tasks to further reduce human effort.
However, these methods have rarely been implemented and
reported in the context of surgical datasets, especially in cataract
surgery datasets. The identification of initial frames to annotate
before the use of any tools or algorithms determines annotation
efficiency. Therefore, in this paper, we chose to prioritize the
development of a method for selecting initial frames to facilitate
the subsequent automated annotation process. We propose a
customized initial frames selection method based on feature
clustering and compare it to commonly used temporal selection
methods. In each method, initial frames from cataract surgery
videos are selected to train a surgical tool detection model.
The model assists in the automated annotation process by
predicting bounding boxes for the surgery video objects in the
remaining frames. Evaluations of these methods are based on
how many edits users need to perform when annotating the
initial frames and how many edits users are expected to perform
to correct all predictions. Additionally, the total annotation cost
for each method is compared. Results indicate that on average,
the proposed cluster-based approach requires the fewest total
edits and exhibits the lowest total annotation cost compared
to conventional methods. These findings highlight a promising
direction for developing a complete application, featuring
streamlined AI-assisted annotation processes for surgical tool
detection tasks.
Yang, Jianming; Hisey, Rebecca; Bierbrier, Joshua; Fichtinger, Gabor; Law, Christine; Holden, Matthew
Frame Selection Methods to Streamline Surgical Video Annotation for Tool Detection Tasks Proceedings Forthcoming
Forthcoming.
@proceedings{nokey,
title = {Frame Selection Methods to Streamline Surgical Video Annotation for Tool Detection Tasks},
author = {Jianming Yang and Rebecca Hisey and Joshua Bierbrier and Gabor Fichtinger and Christine Law and Matthew Holden},
abstract = {—Given the growing volume of surgical data and the
increasing demand for annotation, there is a pressing need to
streamline the annotation process for surgical videos. Previously,
annotation tools for object detection tasks have greatly evolved,
reducing time expense and enhancing ease. There are also many
initial frame selection approaches for Artificial Intelligence
(AI) assisted annotation tasks to further reduce human effort.
However, these methods have rarely been implemented and
reported in the context of surgical datasets, especially in cataract
surgery datasets. The identification of initial frames to annotate
before the use of any tools or algorithms determines annotation
efficiency. Therefore, in this paper, we chose to prioritize the
development of a method for selecting initial frames to facilitate
the subsequent automated annotation process. We propose a
customized initial frames selection method based on feature
clustering and compare it to commonly used temporal selection
methods. In each method, initial frames from cataract surgery
videos are selected to train a surgical tool detection model.
The model assists in the automated annotation process by
predicting bounding boxes for the surgery video objects in the
remaining frames. Evaluations of these methods are based on
how many edits users need to perform when annotating the
initial frames and how many edits users are expected to perform
to correct all predictions. Additionally, the total annotation cost
for each method is compared. Results indicate that on average,
the proposed cluster-based approach requires the fewest total
edits and exhibits the lowest total annotation cost compared
to conventional methods. These findings highlight a promising
direction for developing a complete application, featuring
streamlined AI-assisted annotation processes for surgical tool
detection tasks.},
keywords = {},
pubstate = {forthcoming},
tppubtype = {proceedings}
}
—Given the growing volume of surgical data and the
increasing demand for annotation, there is a pressing need to
streamline the annotation process for surgical videos. Previously,
annotation tools for object detection tasks have greatly evolved,
reducing time expense and enhancing ease. There are also many
initial frame selection approaches for Artificial Intelligence
(AI) assisted annotation tasks to further reduce human effort.
However, these methods have rarely been implemented and
reported in the context of surgical datasets, especially in cataract
surgery datasets. The identification of initial frames to annotate
before the use of any tools or algorithms determines annotation
efficiency. Therefore, in this paper, we chose to prioritize the
development of a method for selecting initial frames to facilitate
the subsequent automated annotation process. We propose a
customized initial frames selection method based on feature
clustering and compare it to commonly used temporal selection
methods. In each method, initial frames from cataract surgery
videos are selected to train a surgical tool detection model.
The model assists in the automated annotation process by
predicting bounding boxes for the surgery video objects in the
remaining frames. Evaluations of these methods are based on
how many edits users need to perform when annotating the
initial frames and how many edits users are expected to perform
to correct all predictions. Additionally, the total annotation cost
for each method is compared. Results indicate that on average,
the proposed cluster-based approach requires the fewest total
edits and exhibits the lowest total annotation cost compared
to conventional methods. These findings highlight a promising
direction for developing a complete application, featuring
streamlined AI-assisted annotation processes for surgical tool
detection tasks.
increasing demand for annotation, there is a pressing need to
streamline the annotation process for surgical videos. Previously,
annotation tools for object detection tasks have greatly evolved,
reducing time expense and enhancing ease. There are also many
initial frame selection approaches for Artificial Intelligence
(AI) assisted annotation tasks to further reduce human effort.
However, these methods have rarely been implemented and
reported in the context of surgical datasets, especially in cataract
surgery datasets. The identification of initial frames to annotate
before the use of any tools or algorithms determines annotation
efficiency. Therefore, in this paper, we chose to prioritize the
development of a method for selecting initial frames to facilitate
the subsequent automated annotation process. We propose a
customized initial frames selection method based on feature
clustering and compare it to commonly used temporal selection
methods. In each method, initial frames from cataract surgery
videos are selected to train a surgical tool detection model.
The model assists in the automated annotation process by
predicting bounding boxes for the surgery video objects in the
remaining frames. Evaluations of these methods are based on
how many edits users need to perform when annotating the
initial frames and how many edits users are expected to perform
to correct all predictions. Additionally, the total annotation cost
for each method is compared. Results indicate that on average,
the proposed cluster-based approach requires the fewest total
edits and exhibits the lowest total annotation cost compared
to conventional methods. These findings highlight a promising
direction for developing a complete application, featuring
streamlined AI-assisted annotation processes for surgical tool
detection tasks.