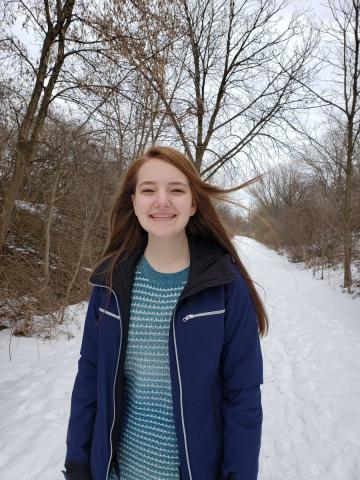
Catherine Austin
Undergraduate Student
School of Computing
Queen’s University
Member from 2021 to present
Biography
Publications
Austin, Catherine; Hisey, Rebecca; O'Driscoll, Olivia; Ungi, Tamas; Fichtinger, Gabor
Using uncertainty quantification to improve reliability of video-based skill assessment metrics in central venous catheterization Journal Article
In: vol. 12466, pp. 84-88, 2023.
@article{fichtinger2023y,
title = {Using uncertainty quantification to improve reliability of video-based skill assessment metrics in central venous catheterization},
author = {Catherine Austin and Rebecca Hisey and Olivia O'Driscoll and Tamas Ungi and Gabor Fichtinger},
url = {https://www.spiedigitallibrary.org/conference-proceedings-of-spie/12466/124660C/Using-uncertainty-quantification-to-improve-reliability-of-video-based-skill/10.1117/12.2654419.short},
year = {2023},
date = {2023-01-01},
volume = {12466},
pages = {84-88},
publisher = {SPIE},
abstract = {Computed-based skill assessment relies on accurate metrics to provide comprehensive feedback to trainees. Improving the accuracy of video-based metrics computed using object detection is generally done by improving the performance of the object detection network, however increasing its performance requires resources that cannot always be obtained. This study aims to improve the accuracy of metrics in central venous catheterization without requiring a high performing object detection network by removing false positive predictions identified using uncertainty quantification. The uncertainty for each bounding box was calculated using an entropy equation. The uncertainties were then compared to an uncertainty threshold computed using the optimal point of a Receiver Operating Characteristic curve. Predictions were removed if the uncertainty fell below the predefined threshold. 50 videos were recorded and …},
keywords = {},
pubstate = {published},
tppubtype = {article}
}
Computed-based skill assessment relies on accurate metrics to provide comprehensive feedback to trainees. Improving the accuracy of video-based metrics computed using object detection is generally done by improving the performance of the object detection network, however increasing its performance requires resources that cannot always be obtained. This study aims to improve the accuracy of metrics in central venous catheterization without requiring a high performing object detection network by removing false positive predictions identified using uncertainty quantification. The uncertainty for each bounding box was calculated using an entropy equation. The uncertainties were then compared to an uncertainty threshold computed using the optimal point of a Receiver Operating Characteristic curve. Predictions were removed if the uncertainty fell below the predefined threshold. 50 videos were recorded and …
Austin, Catherine; Hisey, Rebecca; O'Driscoll, Olivia; Camire, Daenis; Erb, Jason; Howes, Daniel; Ungi, Tamas; Fichtinger, Gabor
Recognizing multiple needle insertion attempts for performance assessment in central venous catheterization training Journal Article
In: vol. 12034, pp. 518-524, 2022.
@article{fichtinger2022r,
title = {Recognizing multiple needle insertion attempts for performance assessment in central venous catheterization training},
author = {Catherine Austin and Rebecca Hisey and Olivia O'Driscoll and Daenis Camire and Jason Erb and Daniel Howes and Tamas Ungi and Gabor Fichtinger},
url = {https://www.spiedigitallibrary.org/conference-proceedings-of-spie/12034/1203428/Recognizing-multiple-needle-insertion-attempts-for-performance-assessment-in-central/10.1117/12.2613190.short},
year = {2022},
date = {2022-01-01},
volume = {12034},
pages = {518-524},
publisher = {SPIE},
abstract = {Purpose
Computer-assisted skill assessment has traditionally been focused on general metrics related to tool motion and usage time. While these metrics are important for an overall evaluation of skill, they do not address critical errors made during the procedure. This study examines the effectiveness of utilizing object detection to quantify the critical error of making multiple needle insertion attempts in central venous catheterization.
Methods
6860 images were annotated with ground truth bounding boxes around the syringe attached to the needle. The images were registered using the location of the phantom, and the bounding boxes from the training set were used to identify the regions where the needle was most likely inserting the phantom. A Faster region-based convolutional neural network was trained to identify the syringe and produce the bounding box location for images in the test set. A needle insertion …},
keywords = {},
pubstate = {published},
tppubtype = {article}
}
Purpose
Computer-assisted skill assessment has traditionally been focused on general metrics related to tool motion and usage time. While these metrics are important for an overall evaluation of skill, they do not address critical errors made during the procedure. This study examines the effectiveness of utilizing object detection to quantify the critical error of making multiple needle insertion attempts in central venous catheterization.
Methods
6860 images were annotated with ground truth bounding boxes around the syringe attached to the needle. The images were registered using the location of the phantom, and the bounding boxes from the training set were used to identify the regions where the needle was most likely inserting the phantom. A Faster region-based convolutional neural network was trained to identify the syringe and produce the bounding box location for images in the test set. A needle insertion …
Computer-assisted skill assessment has traditionally been focused on general metrics related to tool motion and usage time. While these metrics are important for an overall evaluation of skill, they do not address critical errors made during the procedure. This study examines the effectiveness of utilizing object detection to quantify the critical error of making multiple needle insertion attempts in central venous catheterization.
Methods
6860 images were annotated with ground truth bounding boxes around the syringe attached to the needle. The images were registered using the location of the phantom, and the bounding boxes from the training set were used to identify the regions where the needle was most likely inserting the phantom. A Faster region-based convolutional neural network was trained to identify the syringe and produce the bounding box location for images in the test set. A needle insertion …