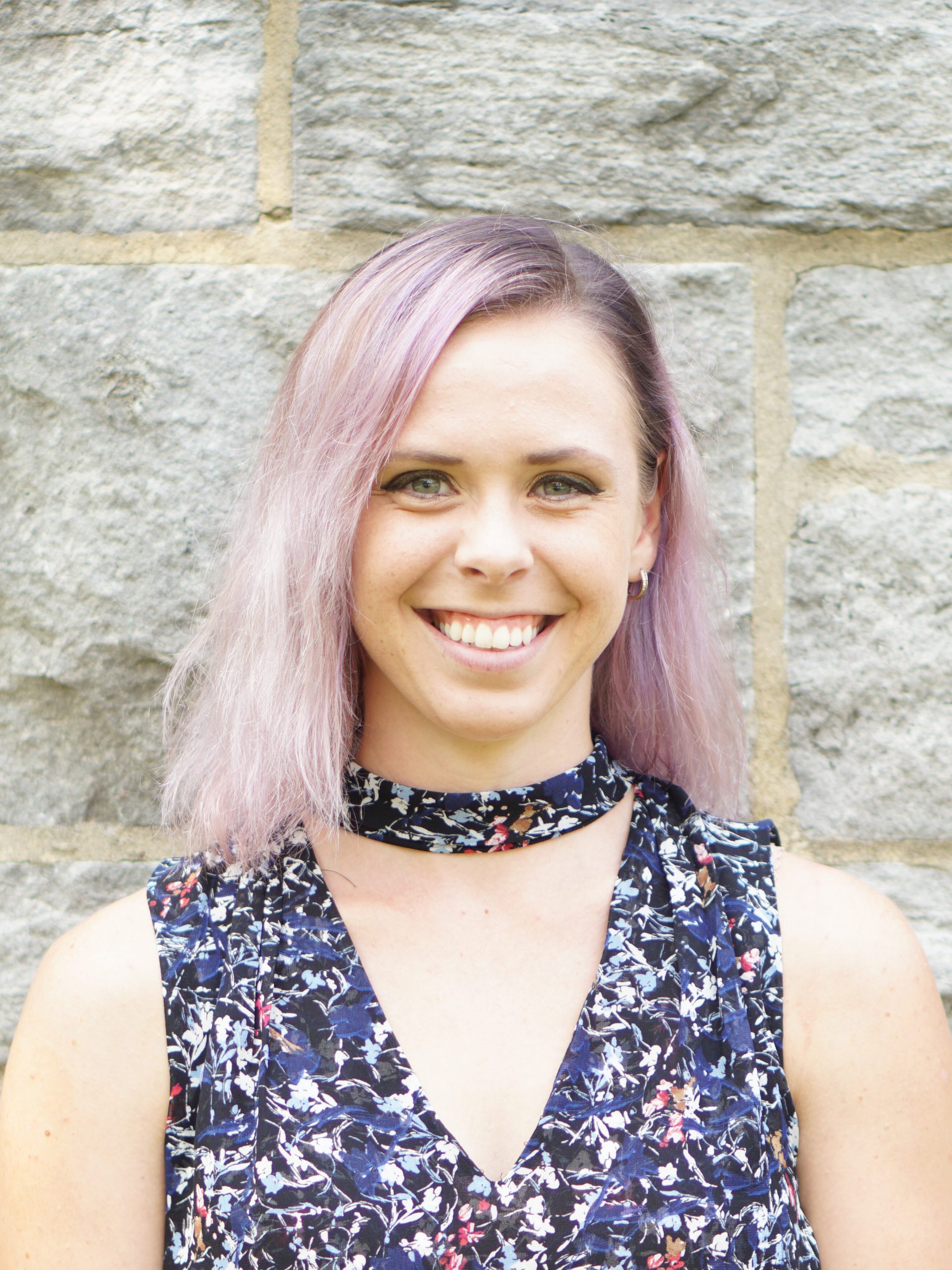
Rebecca Hisey
Biography
Will fill in later
Publications
Ordaz, Daniel Josué Guerra; Cordoba, Magdalena; Delisle, Éolie; Branes, Rocío; Nguyen, Sophie; Daghistani, Waiel Abdulaziz; Mozafarinia, Maryam; Cordoba, Carlos; Maher, Jessica; Dorling, Marisa; Haan, Kirk; Fahad, Danah; Moise, Alexander; Francis, Gizelle; Omar, Youssef; Grose, Elysia; Phillips, Timothy; D'Souza, Alexandra; Datta, Shaishav; Wanzel, Kyle; Bader, Retage Al; Affana, Clementine; Kumar, Ashish; Posel, Nancy; Fleiszer, David; Nguyen, Emily Lan-Vy; Patel, Prachikumari; Irfan, Ahmer; Aubrey, Jason; Coe, Taylor M; Muaddi, Hala; Bucur, Roxana; Rukavina, Nadia; Shwaartz, Chaya; Skaik, Khaled; Elmasry, Wassim; Haseltine, Devon; Bilson, Matthew; Moustafa, Mahmoud; Das, Amrit; Wagner, Maryam; Gomez-Garibello, Carlos; Driad, Cariane; Sonesaksith-Turcotte, Xavier; Sandman, Émilie; Huynh, Lily Trang; Jantchou, Prevost; Nault, Marie-Lyne; Ng, Jasmine; Dhaliwal, Jaskarn; Salim, Henna; Shakeel, Ayesha; Malik, Suffia; Chung, Wiley; Yang, Lucy; Al-Ani, Abdullah; Bondok, Mohamed; Chung, Helen; Gooi, Patrick; Sticca, Giancarlo; Petruccelli, Joseph; Dorion, Dominique; Omar, Yousef Abdelkhalek Saber; Hathi, Kalpesh; Philips, Timothy; Naidoo, Lalenthra; Yang, Xin Yu; Massé, Gabrielle; Tremblay, Jean-François; Vandenbroucke-Menu, Franck; Gervais, Mai-Kim; Letendre, Julien; Jeanmart, Hugues; Lacaille-Ranger, Ariane; Niazi, Farbod; Ahmed, Abrar; Patel, Zeel; Arfaie, Saman; Ma, Crystal; Mikerov, Gregory; Legler, Jack; Steinberg, Emily; Fadel, Elie; Murad, Liam; Biris, Julia; Desgagné, Charles; Colivas, Justine; Noyon, Brandon; Dubrowski, Adam; Patocskai, Érica; Kreutz, Jason; McPhalen, Donald; Temple-Oberle, Claire; Chopra, Sonaina; Dhanoa, Jasmin; Harley, Jason M; Acai, Anita; Keuhl, Amy; Ngo, Quang; Sherbino, Jonathan; Bassilious, Ereny; Bilgic, Elif; Pradhan, Anushka; Volfson, Emily; Tsang, Zackary; Mak, Megan; Hodaie, Mojgan; Peramakumar, Denesh; Hisey, Rebecca; Klosa, Elizabeth; Wong, Aden; Zaza, Farah; Fichtinger, Gabor; Zevin, Boris; Lisondra, James; Gao, Remi; Fung, Albert; Belaiche, Alicia; Piché, Johanie Victoria; Hocini, Adam; Belaiche, Myriam; McNaughton-Filion, Louise; Bouthillier, Constance; Cordoba, Tomas; McEwen, Charlotte; Jaffer, Iqbal; Amin, Faizan; Barsuk, Jeffrey; McGaghie, William; Sibbald, Matthew; Akuffo-Addo, Edgar; Dalson, Jaycie; Agyei, Kwame; Mohsen, Samiha; Yusuf, Safia; Juando-Prats, Clara; Simpson, Jory; Sohi, Gursharan; Giglio, Bianca; Davidovic, Vanja; Yilmaz, Recai; Albeloushi, Abdulmajeed; Alhantoobi, Mohamed; Uthamacumaran, Abicumaran; Lapointe, Jason; Alhaj, Ahmad; Saeedi, Rothaina; Tee, Trisha; Maestro, Rolando Del; Tran, Victoria
C-CASE 2024: Surgical Education Through Innovation: Canadian Conference for the Advancement of Surgical Education, Oct. 17-18, 2024, Toronto, Ontario Journal Article
In: Canadian journal of surgery. Journal canadien de chirurgie, vol. 68, no. 1suppl1, pp. S1-S13, 2025.
@article{ordaz2025b,
title = {C-CASE 2024: Surgical Education Through Innovation: Canadian Conference for the Advancement of Surgical Education, Oct. 17-18, 2024, Toronto, Ontario},
author = {Daniel Josué Guerra Ordaz and Magdalena Cordoba and Éolie Delisle and Rocío Branes and Sophie Nguyen and Waiel Abdulaziz Daghistani and Maryam Mozafarinia and Carlos Cordoba and Jessica Maher and Marisa Dorling and Kirk Haan and Danah Fahad and Alexander Moise and Gizelle Francis and Youssef Omar and Elysia Grose and Timothy Phillips and Alexandra D'Souza and Shaishav Datta and Kyle Wanzel and Retage Al Bader and Clementine Affana and Ashish Kumar and Nancy Posel and David Fleiszer and Emily Lan-Vy Nguyen and Prachikumari Patel and Ahmer Irfan and Jason Aubrey and Taylor M Coe and Hala Muaddi and Roxana Bucur and Nadia Rukavina and Chaya Shwaartz and Khaled Skaik and Wassim Elmasry and Devon Haseltine and Matthew Bilson and Mahmoud Moustafa and Amrit Das and Maryam Wagner and Carlos Gomez-Garibello and Cariane Driad and Xavier Sonesaksith-Turcotte and Émilie Sandman and Lily Trang Huynh and Prevost Jantchou and Marie-Lyne Nault and Jasmine Ng and Jaskarn Dhaliwal and Henna Salim and Ayesha Shakeel and Suffia Malik and Wiley Chung and Lucy Yang and Abdullah Al-Ani and Mohamed Bondok and Helen Chung and Patrick Gooi and Giancarlo Sticca and Joseph Petruccelli and Dominique Dorion and Yousef Abdelkhalek Saber Omar and Kalpesh Hathi and Timothy Philips and Lalenthra Naidoo and Xin Yu Yang and Gabrielle Massé and Jean-François Tremblay and Franck Vandenbroucke-Menu and Mai-Kim Gervais and Julien Letendre and Hugues Jeanmart and Ariane Lacaille-Ranger and Farbod Niazi and Abrar Ahmed and Zeel Patel and Saman Arfaie and Crystal Ma and Gregory Mikerov and Jack Legler and Emily Steinberg and Elie Fadel and Liam Murad and Julia Biris and Charles Desgagné and Justine Colivas and Brandon Noyon and Adam Dubrowski and Érica Patocskai and Jason Kreutz and Donald McPhalen and Claire Temple-Oberle and Sonaina Chopra and Jasmin Dhanoa and Jason M Harley and Anita Acai and Amy Keuhl and Quang Ngo and Jonathan Sherbino and Ereny Bassilious and Elif Bilgic and Anushka Pradhan and Emily Volfson and Zackary Tsang and Megan Mak and Mojgan Hodaie and Denesh Peramakumar and Rebecca Hisey and Elizabeth Klosa and Aden Wong and Farah Zaza and Gabor Fichtinger and Boris Zevin and James Lisondra and Remi Gao and Albert Fung and Alicia Belaiche and Johanie Victoria Piché and Adam Hocini and Myriam Belaiche and Louise McNaughton-Filion and Constance Bouthillier and Tomas Cordoba and Charlotte McEwen and Iqbal Jaffer and Faizan Amin and Jeffrey Barsuk and William McGaghie and Matthew Sibbald and Edgar Akuffo-Addo and Jaycie Dalson and Kwame Agyei and Samiha Mohsen and Safia Yusuf and Clara Juando-Prats and Jory Simpson and Gursharan Sohi and Bianca Giglio and Vanja Davidovic and Recai Yilmaz and Abdulmajeed Albeloushi and Mohamed Alhantoobi and Abicumaran Uthamacumaran and Jason Lapointe and Ahmad Alhaj and Rothaina Saeedi and Trisha Tee and Rolando Del Maestro and Victoria Tran},
year = {2025},
date = {2025-01-01},
journal = {Canadian journal of surgery. Journal canadien de chirurgie},
volume = {68},
number = {1suppl1},
pages = {S1-S13},
abstract = {C-CASE 2024: Surgical Education Through Innovation: Canadian Conference for the Advancement of Surgical Education, Oct. 17-18, 2024, Toronto, Ontario C-CASE 2024: Surgical Education Through Innovation: Canadian Conference for the Advancement of Surgical Education, Oct. 17-18, 2024, Toronto, Ontario Can J Surg. 2025 Feb 6;68(1suppl1):S1-S13. doi: 10.1503/cjs.000225. Print 2025 Jan-Feb. Authors Daniel Josué Guerra Ordaz 1 , Magdalena Cordoba 1 , Éolie Delisle 1 , Rocío Branes 1 , Sophie Nguyen 1 , Waiel Abdulaziz Daghistani 1 , Maryam Mozafarinia 1 , Carlos Cordoba 1 , Jessica Maher 2 , Marisa Dorling 2 , Kirk Haan 2 , Danah Fahad 2 , Alexander Moise 2 , Gizelle Francis 2 , Youssef Omar 2 , Elysia Grose 2 , Timothy Phillips 2 , Alexandra D'Souza 3 , Shaishav Datta 3 , Kyle Wanzel 3 , Retage Al Bader 4 , Clementine Affana 4 , Ashish Kumar 4 , Nancy Posel 5 , David Fleiszer 5 , Emily Lan-Vy …},
keywords = {},
pubstate = {published},
tppubtype = {article}
}
Ordaz, Daniel Josué Guerra; Maher, Jessica; D’Souza, Alexandra; Bader, Retage Al; Posel, Nancy; Nguyen, Emily Lan-Vy; Skaik, Khaled; Driad, Cariane; Ng, Jasmine; Yang, Lucy; Sticca, Giancarlo; Francis, Gizelle; Yang, Xin Yu; Niazi, Farbod; Petruccelli, Joseph; Mikerov, Gregory; Colivas, Justine; Kreutz, Jason; Chopra, Sonaina; Pradhan, Anushka; Volfson, Emily; Peramakumar, Denesh; Patel, Prachikumari; Belaiche, Alicia; Bouthillier, Constance; McEwen, Charlotte; Akuffo-Addo, Edgar; Sohi, Gursharan; Giglio, Bianca; Tran, Victoria; Skakum, Megan; Allen, Rachael; Das, Amrit; McKenna, Alyson; Cordoba, Magdalena; Delisle, Éolie; Branes, Rocío; Nguyen, Sophie; Daghistani, Waiel Abdulaziz; Mozafarinia, Maryam; Cordoba, Carlos; Dorling, Marisa; Haan, Kirk; Fahad, Danah; Moise, Alexander; Omar, Youssef; Grose, Elysia; Phillips, Timothy; Datta, Shaishav; Wanzel, Kyle; Affana, Clementine; Kumar, Ashish; Fleiszer, David; Irfan, Ahmer; Aubrey, Jason; Coe, Taylor M; Muaddi, Hala; Bucur, Roxana; Rukavina, Nadia; Shwaartz, Chaya; Elmasry, Wassim; Haseltine, Devon; Bilson, Matthew; Moustafa, Mahmoud; Wagner, Maryam; Gomez-Garibello, Carlos; Sonesaksith-Turcotte, Xavier; Sandman, Émilie; Huynh, Lily Trang; Jantchou, Prevost; Nault, Marie-Lyne; Dhaliwal, Jaskarn; Salim, Henna; Shakeel, Ayesha; Malik, Suffia; Chung, Wiley; Al-Ani, Abdullah; Bondok, Mohamed; Chung, Helen; Gooi, Patrick; Dorion, Dominique; Omar, Yousef Abdelkhalek Saber; Hathi, Kalpesh; Philips, Timothy; Naidoo, Lalenthra; Massé, Gabrielle; Tremblay, Jean-François; Vandenbroucke-Menu, Franck; Gervais, Mai-Kim; Letendre, Julien; Jeanmart, Hugues; Lacaille-Ranger, Ariane; Ahmed, Abrar; Patel, Zeel; Arfaie, Saman; Ma, Crystal; Legler, Jack; Steinberg, Emily; Fadel, Elie; Murad, Liam; Biris, Julia; Desgagné, Charles; Noyon, Brandon; Dubrowski, Adam; Patocskai, Érica; McPhalen, Donald; Temple-Oberle, Claire; Dhanoa, Jasmin; Harley, Jason M; Acai, Anita; Keuhl, Amy; Ngo, Quang; Sherbino, Jonathan; Bassilious, Ereny; Bilgic, Elif; Tsang, Zackary; Mak, Megan; Hodaie, Mojgan; Hisey, Rebecca; Klosa, Elizabeth; Wong, Aden; Zaza, Farah; Fichtinger, Gabor; Zevin, Boris; Lisondra, James; Gao, Remi; Fung, Albert; Piché, Johanie Victoria; Hocini, Adam; Belaiche, Myriam; McNaughton-Filion, Louise; Cordoba, Tomas; Jaffer, Iqbal; Amin, Faizan; Barsuk, Jeffrey; McGaghie, William; Sibbald, Matthew; Dalson, Jaycie; Agyei, Kwame; Mohsen, Samiha; Yusuf, Safia; Juando-Prats, Clara; Simpson, Jory; Davidovic, Vanja; Yilmaz, Recai; Albeloushi, Abdulmajeed; Alhantoobi, Mohamed; Uthamacumaran, Abicumaran; Lapointe, Jason; Alhaj, Ahmad
C-CASE 2024: Surgical Education Through Innovation01. A 25-year retrospective of Canadian plastic surgery research and its influence: a thorough bibliometric study02 … Journal Article
In: vol. 68, no. 1suppl1, pp. S1-S13, 2025.
@article{ordaz2025,
title = {C-CASE 2024: Surgical Education Through Innovation01. A 25-year retrospective of Canadian plastic surgery research and its influence: a thorough bibliometric study02 …},
author = {Daniel Josué Guerra Ordaz and Jessica Maher and Alexandra D’Souza and Retage Al Bader and Nancy Posel and Emily Lan-Vy Nguyen and Khaled Skaik and Cariane Driad and Jasmine Ng and Lucy Yang and Giancarlo Sticca and Gizelle Francis and Xin Yu Yang and Farbod Niazi and Joseph Petruccelli and Gregory Mikerov and Justine Colivas and Jason Kreutz and Sonaina Chopra and Anushka Pradhan and Emily Volfson and Denesh Peramakumar and Prachikumari Patel and Alicia Belaiche and Constance Bouthillier and Charlotte McEwen and Edgar Akuffo-Addo and Gursharan Sohi and Bianca Giglio and Victoria Tran and Megan Skakum and Rachael Allen and Amrit Das and Alyson McKenna and Magdalena Cordoba and Éolie Delisle and Rocío Branes and Sophie Nguyen and Waiel Abdulaziz Daghistani and Maryam Mozafarinia and Carlos Cordoba and Marisa Dorling and Kirk Haan and Danah Fahad and Alexander Moise and Youssef Omar and Elysia Grose and Timothy Phillips and Shaishav Datta and Kyle Wanzel and Clementine Affana and Ashish Kumar and David Fleiszer and Ahmer Irfan and Jason Aubrey and Taylor M Coe and Hala Muaddi and Roxana Bucur and Nadia Rukavina and Chaya Shwaartz and Wassim Elmasry and Devon Haseltine and Matthew Bilson and Mahmoud Moustafa and Maryam Wagner and Carlos Gomez-Garibello and Xavier Sonesaksith-Turcotte and Émilie Sandman and Lily Trang Huynh and Prevost Jantchou and Marie-Lyne Nault and Jaskarn Dhaliwal and Henna Salim and Ayesha Shakeel and Suffia Malik and Wiley Chung and Abdullah Al-Ani and Mohamed Bondok and Helen Chung and Patrick Gooi and Dominique Dorion and Yousef Abdelkhalek Saber Omar and Kalpesh Hathi and Timothy Philips and Lalenthra Naidoo and Gabrielle Massé and Jean-François Tremblay and Franck Vandenbroucke-Menu and Mai-Kim Gervais and Julien Letendre and Hugues Jeanmart and Ariane Lacaille-Ranger and Abrar Ahmed and Zeel Patel and Saman Arfaie and Crystal Ma and Jack Legler and Emily Steinberg and Elie Fadel and Liam Murad and Julia Biris and Charles Desgagné and Brandon Noyon and Adam Dubrowski and Érica Patocskai and Donald McPhalen and Claire Temple-Oberle and Jasmin Dhanoa and Jason M Harley and Anita Acai and Amy Keuhl and Quang Ngo and Jonathan Sherbino and Ereny Bassilious and Elif Bilgic and Zackary Tsang and Megan Mak and Mojgan Hodaie and Rebecca Hisey and Elizabeth Klosa and Aden Wong and Farah Zaza and Gabor Fichtinger and Boris Zevin and James Lisondra and Remi Gao and Albert Fung and Johanie Victoria Piché and Adam Hocini and Myriam Belaiche and Louise McNaughton-Filion and Tomas Cordoba and Iqbal Jaffer and Faizan Amin and Jeffrey Barsuk and William McGaghie and Matthew Sibbald and Jaycie Dalson and Kwame Agyei and Samiha Mohsen and Safia Yusuf and Clara Juando-Prats and Jory Simpson and Vanja Davidovic and Recai Yilmaz and Abdulmajeed Albeloushi and Mohamed Alhantoobi and Abicumaran Uthamacumaran and Jason Lapointe and Ahmad Alhaj},
year = {2025},
date = {2025-01-01},
volume = {68},
number = {1suppl1},
pages = {S1-S13},
publisher = {Canadian Journal of Surgery},
abstract = {Background:
Bibliometric analysis is used to assess and interpret the academic output and impact within a specific field. We aimed to measure the quantity and quality of plastic surgery research conducted by Canadian authors from 1999 to 2023.
Methods:
An extensive bibliometric analysis was carried out using the Web of Science Core Collection, retrieving data from 60 leading plastic surgery journals, focusing on original articles and reviews published between 1999 and 2023. The InCites Benchmarking & Analytics platform was used to evaluate the quantity and quality of the publications. The quality was assessed using 2 main metrics: category-normalized citation impact (CNCI) and the percentage of publications in the top quartile of journals based on impact factors. We employed VOSviewer to visualize notable keywords and map collaborative relationships among universities over various periods.
Results …},
keywords = {},
pubstate = {published},
tppubtype = {article}
}
Bibliometric analysis is used to assess and interpret the academic output and impact within a specific field. We aimed to measure the quantity and quality of plastic surgery research conducted by Canadian authors from 1999 to 2023.
Methods:
An extensive bibliometric analysis was carried out using the Web of Science Core Collection, retrieving data from 60 leading plastic surgery journals, focusing on original articles and reviews published between 1999 and 2023. The InCites Benchmarking & Analytics platform was used to evaluate the quantity and quality of the publications. The quality was assessed using 2 main metrics: category-normalized citation impact (CNCI) and the percentage of publications in the top quartile of journals based on impact factors. We employed VOSviewer to visualize notable keywords and map collaborative relationships among universities over various periods.
Results …
Kim, Andrew S.; Yeung, Chris; Szabo, Robert; Sunderland, Kyle; Hisey, Rebecca; Morton, David; Kikinis, Ron; Diao, Babacar; Mousavi, Parvin; Ungi, Tamas; Fichtinger, Gabor
SPIE, 2024.
@proceedings{Kim2024,
title = {Percutaneous nephrostomy needle guidance using real-time 3D anatomical visualization with live ultrasound segmentation},
author = {Andrew S. Kim and Chris Yeung and Robert Szabo and Kyle Sunderland and Rebecca Hisey and David Morton and Ron Kikinis and Babacar Diao and Parvin Mousavi and Tamas Ungi and Gabor Fichtinger},
editor = {Maryam E. Rettmann and Jeffrey H. Siewerdsen},
doi = {10.1117/12.3006533},
year = {2024},
date = {2024-03-29},
urldate = {2024-03-29},
publisher = {SPIE},
abstract = {
PURPOSE: Percutaneous nephrostomy is a commonly performed procedure to drain urine to provide relief in patients with hydronephrosis. Conventional percutaneous nephrostomy needle guidance methods can be difficult, expensive, or not portable. We propose an open-source real-time 3D anatomical visualization aid for needle guidance with live ultrasound segmentation and 3D volume reconstruction using free, open-source software. METHODS: Basic hydronephrotic kidney phantoms were created, and recordings of these models were manually segmented and used to train a deep learning model that makes live segmentation predictions to perform live 3D volume reconstruction of the fluid-filled cavity. Participants performed 5 needle insertions with the visualization aid and 5 insertions with ultrasound needle guidance on a kidney phantom in randomized order, and these were recorded. Recordings of the trials were analyzed for needle tip distance to the center of the target calyx, needle insertion time, and success rate. Participants also completed a survey on their experience. RESULTS: Using the visualization aid showed significantly higher accuracy, while needle insertion time and success rate were not statistically significant at our sample size. Participants mostly responded positively to the visualization aid, and 80% found it easier to use than ultrasound needle guidance. CONCLUSION: We found that our visualization aid produced increased accuracy and an overall positive experience. We demonstrated that our system is functional and stable and believe that the workflow with this system can be applied to other procedures. This visualization aid system is effective on phantoms and is ready for translation with clinical data.},
keywords = {},
pubstate = {published},
tppubtype = {proceedings}
}
PURPOSE: Percutaneous nephrostomy is a commonly performed procedure to drain urine to provide relief in patients with hydronephrosis. Conventional percutaneous nephrostomy needle guidance methods can be difficult, expensive, or not portable. We propose an open-source real-time 3D anatomical visualization aid for needle guidance with live ultrasound segmentation and 3D volume reconstruction using free, open-source software. METHODS: Basic hydronephrotic kidney phantoms were created, and recordings of these models were manually segmented and used to train a deep learning model that makes live segmentation predictions to perform live 3D volume reconstruction of the fluid-filled cavity. Participants performed 5 needle insertions with the visualization aid and 5 insertions with ultrasound needle guidance on a kidney phantom in randomized order, and these were recorded. Recordings of the trials were analyzed for needle tip distance to the center of the target calyx, needle insertion time, and success rate. Participants also completed a survey on their experience. RESULTS: Using the visualization aid showed significantly higher accuracy, while needle insertion time and success rate were not statistically significant at our sample size. Participants mostly responded positively to the visualization aid, and 80% found it easier to use than ultrasound needle guidance. CONCLUSION: We found that our visualization aid produced increased accuracy and an overall positive experience. We demonstrated that our system is functional and stable and believe that the workflow with this system can be applied to other procedures. This visualization aid system is effective on phantoms and is ready for translation with clinical data.
d'Albenzio, Gabriella; Hisey, Rebecca; Srikanthan, Dilakshan; Ungi, Tamas; Lasso, Andras; Aghayan, Davit; Fichtinger, Gabor; Palomar, Rafael
Using NURBS for virtual resections in liver surgery planning: a comparative usability study Journal Article
In: vol. 12927, pp. 235-241, 2024.
@article{fichtinger2024f,
title = {Using NURBS for virtual resections in liver surgery planning: a comparative usability study},
author = {Gabriella d'Albenzio and Rebecca Hisey and Dilakshan Srikanthan and Tamas Ungi and Andras Lasso and Davit Aghayan and Gabor Fichtinger and Rafael Palomar},
url = {https://www.spiedigitallibrary.org/conference-proceedings-of-spie/12927/129270Z/Using-NURBS-for-virtual-resections-in-liver-surgery-planning/10.1117/12.3006486.short},
year = {2024},
date = {2024-01-01},
volume = {12927},
pages = {235-241},
publisher = {SPIE},
abstract = {PURPOSE
Accurate preoperative planning is crucial for liver resection surgery due to the complex anatomical structures and variations among patients. The need of virtual resections utilizing deformable surfaces presents a promising approach for effective liver surgery planning. However, the range of available surface definitions poses the question of which definition is most appropriate.
METHODS
The study compares the use of NURBS and B´ezier surfaces for the definition of virtual resections through a usability study, where 25 participants (19 biomedical researchers and 6 liver surgeons) completed tasks using varying numbers of control points driving surface deformations and different surface types. Specifically, participants aim to perform virtual liver resections using 16 and 9 control points for NURBS and B´ezier surfaces. The goal is to assess whether they can attain an optimal resection plan, effectively …},
keywords = {},
pubstate = {published},
tppubtype = {article}
}
Accurate preoperative planning is crucial for liver resection surgery due to the complex anatomical structures and variations among patients. The need of virtual resections utilizing deformable surfaces presents a promising approach for effective liver surgery planning. However, the range of available surface definitions poses the question of which definition is most appropriate.
METHODS
The study compares the use of NURBS and B´ezier surfaces for the definition of virtual resections through a usability study, where 25 participants (19 biomedical researchers and 6 liver surgeons) completed tasks using varying numbers of control points driving surface deformations and different surface types. Specifically, participants aim to perform virtual liver resections using 16 and 9 control points for NURBS and B´ezier surfaces. The goal is to assess whether they can attain an optimal resection plan, effectively …
Hisey, Rebecca; Ndiaye, Fatou Bintou; Sunderland, Kyle; Seck, Idrissa; Mbaye, Moustapha; Keita, Mohamed; Diahame, Mamadou; Kikinis, Ron; Diao, Babacar; Fichtinger, Gabor; Camara, Mamadou
Feasibility of video-based skill assessment for percutaneous nephrostomy training in Senegal Journal Article
In: 2024.
@article{hisey2024,
title = {Feasibility of video-based skill assessment for percutaneous nephrostomy training in Senegal},
author = {Rebecca Hisey and Fatou Bintou Ndiaye and Kyle Sunderland and Idrissa Seck and Moustapha Mbaye and Mohamed Keita and Mamadou Diahame and Ron Kikinis and Babacar Diao and Gabor Fichtinger and Mamadou Camara},
year = {2024},
date = {2024-01-01},
keywords = {},
pubstate = {published},
tppubtype = {article}
}
Yang, Jianming; Hisey, Rebecca; Bierbrier, Joshua; Law, Christine; Fichtinger, Gabor; Holden, Matthew
Frame Selection Methods to Streamline Surgical Video Annotation for Tool Detection Tasks Journal Article
In: pp. 892-898, 2024.
@article{yang2024,
title = {Frame Selection Methods to Streamline Surgical Video Annotation for Tool Detection Tasks},
author = {Jianming Yang and Rebecca Hisey and Joshua Bierbrier and Christine Law and Gabor Fichtinger and Matthew Holden},
year = {2024},
date = {2024-01-01},
pages = {892-898},
publisher = {IEEE},
abstract = {Given the growing volume of surgical data and the increasing demand for annotation, there is a pressing need to streamline the annotation process for surgical videos. Previously, annotation tools for object detection tasks have greatly evolved, reducing time expense and enhancing ease. There are also many initial frame selection approaches for Artificial Intelligence (AI) assisted annotation tasks to further reduce human effort. However, these methods have rarely been implemented and reported in the context of surgical datasets, especially in cataract surgery datasets. The identification of initial frames to annotate before the use of any tools or algorithms determines annotation efficiency. Therefore, in this paper, we chose to prioritize the development of a method for selecting initial frames to facilitate the subsequent automated annotation process. We propose a customized initial frames selection method based on …},
keywords = {},
pubstate = {published},
tppubtype = {article}
}
Hisey, Rebecca; Ndiaye, Fatou Bintou; Sunderland, Kyle; Seck, Idrissa; Mbaye, Moustapha; Keita, Mohammed; Diahame, Mamadou; Kikinis, Ron; Diao, Babacar; Fichtinger, Gabor; Camara, Mamadou
Feasibility of video‐based skill assessment for percutaneous nephrostomy training in Senegal Journal Article
In: Healthcare Technology Letters, 2024.
@article{hisey2024a,
title = {Feasibility of video‐based skill assessment for percutaneous nephrostomy training in Senegal},
author = {Rebecca Hisey and Fatou Bintou Ndiaye and Kyle Sunderland and Idrissa Seck and Moustapha Mbaye and Mohammed Keita and Mamadou Diahame and Ron Kikinis and Babacar Diao and Gabor Fichtinger and Mamadou Camara},
year = {2024},
date = {2024-01-01},
journal = {Healthcare Technology Letters},
abstract = {Percutaneous nephrostomy can be an effective means of preventing irreparable renal damage from obstructive renal disease thereby providing patients with more time to access treatment to remove the source of the blockage. In sub‐Saharan Africa, where there is limited access to treatments such as dialysis and transplantation, a nephrostomy can be life‐saving. Training this procedure in simulation can allow trainees to develop their technical skills without risking patient safety, but still requires an ex‐pert observer to provide performative feedback. In this study, the feasibility of using video as an accessible method to assess skill in simulated percutaneous nephrostomy is evaluated. Six novice urology residents and six expert urologists from Ouakam Military Hospital in Dakar, Senegal performed 4 nephrostomies each using the setup. Motion‐based metrics were computed for each trial from the predicted bounding …},
keywords = {},
pubstate = {published},
tppubtype = {article}
}
Klosa, Elizabeth; Hisey, Rebecca; Hashtrudi-Zaad, Kian; Zevin, Boris; Ungi, Tamas; Fichtinger, Gabor
Comparing methods of identifying tissues for workflow recognition of simulated open hernia repair Conference
2023.
@conference{nokey,
title = {Comparing methods of identifying tissues for workflow recognition of simulated open hernia repair},
author = {Elizabeth Klosa and Rebecca Hisey and Kian Hashtrudi-Zaad and Boris Zevin and Tamas Ungi and Gabor Fichtinger},
url = {https://imno.ca/sites/default/files/ImNO2023Proceedings.pdf},
year = {2023},
date = {2023-03-24},
urldate = {2024-03-24},
keywords = {},
pubstate = {published},
tppubtype = {conference}
}
March, Lucas; Rodgers, Jessica R.; Hisey, Rebecca; Jamzad, Amoon; Santilli, AML; McKay, D; Rudan, JF; Kaufmann, M; Ren, KYM; Fichtinger, G; Mousavi, P
Cautery tool state detection using deep learning on intraoperative surgery videos Journal Article
In: vol. 12466, pp. 89-95, 2023.
@article{fichtinger2023o,
title = {Cautery tool state detection using deep learning on intraoperative surgery videos},
author = {Lucas March and Jessica R. Rodgers and Rebecca Hisey and Amoon Jamzad and AML Santilli and D McKay and JF Rudan and M Kaufmann and KYM Ren and G Fichtinger and P Mousavi},
url = {https://www.spiedigitallibrary.org/conference-proceedings-of-spie/12466/124660D/Cautery-tool-state-detection-using-deep-learning-on-intraoperative-surgery/10.1117/12.2654234.short},
year = {2023},
date = {2023-01-01},
urldate = {2023-01-01},
volume = {12466},
pages = {89-95},
publisher = {SPIE},
abstract = {Treatment for Basal Cell Carcinoma (BCC) includes an excisional surgery to remove cancerous tissues, using a cautery tool to make burns along a defined resection margin around the tumor. Margin evaluation occurs post-surgically, requiring repeat surgery if positive margins are detected. Rapid Evaporative Ionization Mass Spectrometry (REIMS) can help distinguish healthy and cancerous tissue but does not provide spatial information about the cautery tool location where the spectra are acquired. We propose using intraoperative surgical video recordings and deep learning to provide surgeons with guidance to locate sites of potential positive margins. Frames from 14 intraoperative videos of BCC surgery were extracted and used to train a sequence of networks. The first network extracts frames showing surgery in-progress, then, an object detection network localizes the cautery tool and resection margin. Finally …},
keywords = {},
pubstate = {published},
tppubtype = {article}
}
Austin, Catherine; Hisey, Rebecca; O'Driscoll, Olivia; Ungi, Tamas; Fichtinger, Gabor
Using uncertainty quantification to improve reliability of video-based skill assessment metrics in central venous catheterization Journal Article
In: vol. 12466, pp. 84-88, 2023.
@article{fichtinger2023y,
title = {Using uncertainty quantification to improve reliability of video-based skill assessment metrics in central venous catheterization},
author = {Catherine Austin and Rebecca Hisey and Olivia O'Driscoll and Tamas Ungi and Gabor Fichtinger},
url = {https://www.spiedigitallibrary.org/conference-proceedings-of-spie/12466/124660C/Using-uncertainty-quantification-to-improve-reliability-of-video-based-skill/10.1117/12.2654419.short},
year = {2023},
date = {2023-01-01},
volume = {12466},
pages = {84-88},
publisher = {SPIE},
abstract = {Computed-based skill assessment relies on accurate metrics to provide comprehensive feedback to trainees. Improving the accuracy of video-based metrics computed using object detection is generally done by improving the performance of the object detection network, however increasing its performance requires resources that cannot always be obtained. This study aims to improve the accuracy of metrics in central venous catheterization without requiring a high performing object detection network by removing false positive predictions identified using uncertainty quantification. The uncertainty for each bounding box was calculated using an entropy equation. The uncertainties were then compared to an uncertainty threshold computed using the optimal point of a Receiver Operating Characteristic curve. Predictions were removed if the uncertainty fell below the predefined threshold. 50 videos were recorded and …},
keywords = {},
pubstate = {published},
tppubtype = {article}
}
Klosa, Elizabeth; Hisey, Rebecca; Hashtrudi-Zaad, Kian; Zevin, Boris; Ungi, Tamas; Fichtinger, Gabor
Identifying tool-tissue interactions to distinguish steps in simulated open inguinal hernia repair Journal Article
In: vol. 12466, pp. 479-486, 2023.
@article{fichtinger2023s,
title = {Identifying tool-tissue interactions to distinguish steps in simulated open inguinal hernia repair},
author = {Elizabeth Klosa and Rebecca Hisey and Kian Hashtrudi-Zaad and Boris Zevin and Tamas Ungi and Gabor Fichtinger},
url = {https://www.spiedigitallibrary.org/conference-proceedings-of-spie/12466/1246620/Identifying-tool-tissue-interactions-to-distinguish-steps-in-simulated-open/10.1117/12.2654394.short},
year = {2023},
date = {2023-01-01},
volume = {12466},
pages = {479-486},
publisher = {SPIE},
abstract = {As medical education adopts a competency-based training approach, assessment of skills and timely provision of formative feedback is required. Provision of such assessment and feedback places a substantial time burden on surgeons. To reduce this time burden, we look to develop a computer-assisted training platform to provide both instruction and feedback to residents learning open Inguinal Hernia Repairs (IHR). To provide feedback on residents’ technical skills, we must first find a method of workflow recognition of the IHR. We thus aim to recognize and distinguish between workflow steps of an open IHR based on the presence and frequencies of different tool-tissue interactions occurring during each step. Based on ground truth tissue segmentations and tool bounding boxes, we identify the visible tissues within a bounding box. This provides an estimation of which tissues a tool is interacting with. The …},
keywords = {},
pubstate = {published},
tppubtype = {article}
}
Hashtrudi-Zaad, Kian; Hisey, Rebecca; Klosa, Elizabeth; Zevin, Boris; Ungi, Tamas; Fichtinger, Gabor
Using object detection for surgical tool recognition in simulated open inguinal hernia repair surgery Journal Article
In: vol. 12466, pp. 96-101, 2023.
@article{fichtinger2023p,
title = {Using object detection for surgical tool recognition in simulated open inguinal hernia repair surgery},
author = {Kian Hashtrudi-Zaad and Rebecca Hisey and Elizabeth Klosa and Boris Zevin and Tamas Ungi and Gabor Fichtinger},
url = {https://www.spiedigitallibrary.org/conference-proceedings-of-spie/12466/124660E/Using-object-detection-for-surgical-tool-recognition-in-simulated-open/10.1117/12.2654393.short},
year = {2023},
date = {2023-01-01},
volume = {12466},
pages = {96-101},
publisher = {SPIE},
abstract = {Following the shift from time-based medical education to a competency-based approach, a computer-assisted training platform would help relieve some of the new time burden placed on physicians. A vital component of these platforms is the computation of competency metrics which are based on surgical tool motion. Recognizing the class and motion of surgical tools is one step in the development of a training platform. Object detection can achieve tool recognition. While previous literature has reported on tool recognition in minimally invasive surgeries, open surgeries have not received the same attention. Open Inguinal Hernia Repair (OIHR), a common surgery that general surgery residents must learn, is an example of such surgeries. We present a method for object detection to recognize surgical tools in simulated OIHR. Images were extracted from six video recordings of OIHR performed on phantoms. Tools …},
keywords = {},
pubstate = {published},
tppubtype = {article}
}
Ndiaye, Fatou Bintou; Groves, Leah; Hisey, Rebecca; Ungi, Tamas; Diop, Idy; Mousavi, Parvin; Fichtinger, Gabor; Camara, Mamadou Samba
Desing and realization of a computer-assisted nephrostomy guidance system Journal Article
In: pp. 1-6, 2023.
@article{fichtinger2023l,
title = {Desing and realization of a computer-assisted nephrostomy guidance system},
author = {Fatou Bintou Ndiaye and Leah Groves and Rebecca Hisey and Tamas Ungi and Idy Diop and Parvin Mousavi and Gabor Fichtinger and Mamadou Samba Camara},
url = {https://ieeexplore.ieee.org/abstract/document/10253146/},
year = {2023},
date = {2023-01-01},
pages = {1-6},
publisher = {IEEE},
abstract = {Background and purpose
Nowadays, computerized nephrostomy techniques exist. Although relatively safe, several factors make it difficult for inexperienced users. A computer-assisted nephrostomy guidance system has been studied to increase the success rate of this intervention and reduce the work and difficulties encountered by the actors.
Methods
To design the system, two methods will be studied. Following this study, this system was designed based on method 2. SmartSysNephro is composed of a hardware part whose manipulations made by the user are visualized and assisted by the computer. This nephrostomy procedure that the user simulates is monitored by webcam. Using the data from this Intel Real Sense webcam, allowed to propose a CNN YOLO model.
Results
The results obtained show that the objectives set have been achieved globally. The SmartSysNephro system gives real time warning …},
keywords = {},
pubstate = {published},
tppubtype = {article}
}
Nowadays, computerized nephrostomy techniques exist. Although relatively safe, several factors make it difficult for inexperienced users. A computer-assisted nephrostomy guidance system has been studied to increase the success rate of this intervention and reduce the work and difficulties encountered by the actors.
Methods
To design the system, two methods will be studied. Following this study, this system was designed based on method 2. SmartSysNephro is composed of a hardware part whose manipulations made by the user are visualized and assisted by the computer. This nephrostomy procedure that the user simulates is monitored by webcam. Using the data from this Intel Real Sense webcam, allowed to propose a CNN YOLO model.
Results
The results obtained show that the objectives set have been achieved globally. The SmartSysNephro system gives real time warning …
Klosa, Elizabeth; Hisey, Rebecca; Nazari, Tahmina; Wiggers, Theo; Zevin, Boris; Ungi, Tamas; Fichtinger, Gabor
Identifying tissues for task recognition in training of open inguinal hernia repairs Conference
Imaging Network of Ontario Symposium, 2022.
@conference{Klosa2022b,
title = {Identifying tissues for task recognition in training of open inguinal hernia repairs},
author = {Elizabeth Klosa and Rebecca Hisey and Tahmina Nazari and Theo Wiggers and Boris Zevin and Tamas Ungi and Gabor Fichtinger},
url = {https://labs.cs.queensu.ca/perklab/wp-content/uploads/sites/3/2024/03/Klosa2022b.pdf},
year = {2022},
date = {2022-02-01},
urldate = {2022-02-01},
booktitle = {Imaging Network of Ontario Symposium},
keywords = {},
pubstate = {published},
tppubtype = {conference}
}
O’Driscoll, Olivia; Hisey, Rebecca; Holden, M.; Camire, Daenis; Erb, Jason; Howes, Daniel; Ungi, Tamas; Fichtinger, Gabor
Feasibility of using object detection for performance assessment in central venous catherization Conference
Imaging Network of Ontario Symposium, 2022.
@conference{ODriscoll2022b,
title = {Feasibility of using object detection for performance assessment in central venous catherization},
author = {Olivia O’Driscoll and Rebecca Hisey and M. Holden and Daenis Camire and Jason Erb and Daniel Howes and Tamas Ungi and Gabor Fichtinger},
url = {https://labs.cs.queensu.ca/perklab/wp-content/uploads/sites/3/2024/02/ODriscoll2021b.pdf},
year = {2022},
date = {2022-02-01},
urldate = {2022-02-01},
booktitle = {Imaging Network of Ontario Symposium},
keywords = {},
pubstate = {published},
tppubtype = {conference}
}
O’Driscoll, Olivia; Hisey, Rebecca; Holden, M.; Camire, Daenis; Erb, Jason; Howes, Daniel; Ungi, Tamas; Fichtinger, Gabor
Feasibility of object detection for skill assessment in central venous catheterization Conference
SPIE Medical Imaging, SPIE Medical Imaging SPIE Medical Imaging, San Diego, 2022.
@conference{ODriscoll2022a,
title = {Feasibility of object detection for skill assessment in central venous catheterization},
author = {Olivia O’Driscoll and Rebecca Hisey and M. Holden and Daenis Camire and Jason Erb and Daniel Howes and Tamas Ungi and Gabor Fichtinger},
url = {https://labs.cs.queensu.ca/perklab/wp-content/uploads/sites/3/2024/02/ODriscoll2022a.pdf},
year = {2022},
date = {2022-02-01},
urldate = {2022-02-01},
booktitle = {SPIE Medical Imaging},
publisher = {SPIE Medical Imaging},
address = {San Diego},
organization = {SPIE Medical Imaging},
abstract = {<p><strong>Purpose: </strong>Computer-assisted surgical skill assessment methods have traditionally relied on tracking tool motion with physical sensors. These tracking systems can be expensive, bulky, and impede tool function. Recent advances in object detection networks have made it possible to quantify tool motion using only a camera. These advances open the door for a low-cost alternative to current physical tracking systems for surgical skill assessment. This study determines the feasibility of using metrics computed with object detection by comparing them to widely accepted metrics computed using traditional tracking methods in central venous catheterization. <strong>Methods:</strong> Both video and tracking data were recorded from participants performing central venous catheterization on a venous access phantom. A Faster Region-Based Convolutional Neural Network was trained to recognize the ultrasound probe and syringe on the video data. Tracking-based metrics were computed using the Perk Tutor extension of 3D Slicer. The path length and usage time for each tool were then computed using both the video and tracking data. The metrics from object detection and tracking were compared using Spearman rank correlation. <strong>Results: </strong>The path lengths had a rank correlation coefficient of 0.22 for the syringe (p<0.03) and 0.35 (p<0.001) for the ultrasound probe. For the usage times, the correlation coefficient was 0.37 (p<0.001) for the syringe and 0.34 (p<0.001) for the ultrasound probe. <strong>Conclusions</strong>: The video-based metrics correlated significantly with the tracked metrics, suggesting that object detection could be a feasible skill assessment method for central venous catheterization.</p>},
keywords = {},
pubstate = {published},
tppubtype = {conference}
}
Klosa, Elizabeth; Hisey, Rebecca; Nazari, Tahmina; Wiggers, Theo; Zevin, Boris; Ungi, Tamas; Fichtinger, Gabor
Tissue segmentation for workflow recognition in open inguinal hernia repair training Conference
SPIE Medical Imaging, SPIE Medical Imaging SPIE Medical Imaging, San Diego, 2022.
@conference{Klosa2022a,
title = {Tissue segmentation for workflow recognition in open inguinal hernia repair training},
author = {Elizabeth Klosa and Rebecca Hisey and Tahmina Nazari and Theo Wiggers and Boris Zevin and Tamas Ungi and Gabor Fichtinger},
url = {https://labs.cs.queensu.ca/perklab/wp-content/uploads/sites/3/2024/02/Klosa2022a.pdf},
year = {2022},
date = {2022-02-01},
urldate = {2022-02-01},
booktitle = {SPIE Medical Imaging},
publisher = {SPIE Medical Imaging},
address = {San Diego},
organization = {SPIE Medical Imaging},
abstract = {PURPOSE: As medical education adopts a competency-based training method, experts are spending substantial amounts of time instructing and assessing trainees’ competence. In this study, we look to develop a computer-assisted training platform that can provide instruction and assessment of open inguinal hernia repairs without needing an expert observer. We recognize workflow tasks based on the tool-tissue interactions, suggesting that we first need a method to identify tissues. This study aims to train a neural network in identifying tissues in a low-cost phantom as we work towards identifying the tool-tissue interactions needed for task recognition. METHODS: Eight simulated tissues were segmented throughout five videos from experienced surgeons who performed open inguinal hernia repairs on phantoms. A U-Net was trained using leave-one-user-out cross validation. The average F-score, false positive rate and false negative rate were calculated for each tissue to evaluate the U-Net’s performance. RESULTS: Higher F-scores and lower false negative and positive rates were recorded for the skin, hernia sac, spermatic cord, and nerves, while slightly lower metrics were recorded for the subcutaneous tissue, Scarpa’s fascia, external oblique aponeurosis and superficial epigastric vessels. CONCLUSION: The U-Net performed better in recognizing tissues that were relatively larger in size and more prevalent, while struggling to recognize smaller tissues only briefly visible. Since workflow recognition does not require perfect segmentation, we believe our U-Net is sufficient in recognizing the tissues of an inguinal hernia repair phantom. Future studies will explore combining our segmentation U-Net with tool detection as we work towards workflow recognition.},
keywords = {},
pubstate = {published},
tppubtype = {conference}
}
Austin, Catherine; Hisey, Rebecca; O'Driscoll, Olivia; Camire, Daenis; Erb, Jason; Howes, Daniel; Ungi, Tamas; Fichtinger, Gabor
Recognizing multiple needle insertion attempts for performance assessment in central venous catheterization training Journal Article
In: vol. 12034, pp. 518-524, 2022.
@article{fichtinger2022r,
title = {Recognizing multiple needle insertion attempts for performance assessment in central venous catheterization training},
author = {Catherine Austin and Rebecca Hisey and Olivia O'Driscoll and Daenis Camire and Jason Erb and Daniel Howes and Tamas Ungi and Gabor Fichtinger},
url = {https://www.spiedigitallibrary.org/conference-proceedings-of-spie/12034/1203428/Recognizing-multiple-needle-insertion-attempts-for-performance-assessment-in-central/10.1117/12.2613190.short},
year = {2022},
date = {2022-01-01},
volume = {12034},
pages = {518-524},
publisher = {SPIE},
abstract = {Purpose
Computer-assisted skill assessment has traditionally been focused on general metrics related to tool motion and usage time. While these metrics are important for an overall evaluation of skill, they do not address critical errors made during the procedure. This study examines the effectiveness of utilizing object detection to quantify the critical error of making multiple needle insertion attempts in central venous catheterization.
Methods
6860 images were annotated with ground truth bounding boxes around the syringe attached to the needle. The images were registered using the location of the phantom, and the bounding boxes from the training set were used to identify the regions where the needle was most likely inserting the phantom. A Faster region-based convolutional neural network was trained to identify the syringe and produce the bounding box location for images in the test set. A needle insertion …},
keywords = {},
pubstate = {published},
tppubtype = {article}
}
Computer-assisted skill assessment has traditionally been focused on general metrics related to tool motion and usage time. While these metrics are important for an overall evaluation of skill, they do not address critical errors made during the procedure. This study examines the effectiveness of utilizing object detection to quantify the critical error of making multiple needle insertion attempts in central venous catheterization.
Methods
6860 images were annotated with ground truth bounding boxes around the syringe attached to the needle. The images were registered using the location of the phantom, and the bounding boxes from the training set were used to identify the regions where the needle was most likely inserting the phantom. A Faster region-based convolutional neural network was trained to identify the syringe and produce the bounding box location for images in the test set. A needle insertion …
Lee, H. Y.; Hisey, Rebecca; Holden, Matthew; Liu, John; Ungi, Tamas; Fichtinger, Gabor; Law, Christine
Evaluating Faster R-CNN for cataract surgery tool detection using microscopy video Conference
Imaging Network of Ontario Symposium , 2022.
@conference{Lee2022a,
title = {Evaluating Faster R-CNN for cataract surgery tool detection using microscopy video},
author = {H. Y. Lee and Rebecca Hisey and Matthew Holden and John Liu and Tamas Ungi and Gabor Fichtinger and Christine Law},
year = {2022},
date = {2022-01-01},
urldate = {2022-01-01},
booktitle = {Imaging Network of Ontario Symposium
},
abstract = {<p>Introduction: Traditional methods of cataract surgery skill assessment rely on human expert supervision. This exposes the trainee to interobserver variability and inconsistent feedback. Alternative measures such as sensorbased instrument motion analysis promise objective assessment [1]. However, sensor-based systems are logistically complicated and expensive to obtain. Previous studies have demonstrated a strong correlation between sensor-based metrics and two-dimensional motion metrics obtained from object detection [2]. Reliable object detection is the foundation for computing such performance metrics. Therefore, the objective of this study is to evaluate the performance of an object detection network, namely Faster Region-Based Convolutional Neural Network (FRCNN), in recognition of cataract surgery tools in microscopy video. Methods: Microscope video was recorded for 25 trials of cataract surgery on an artificial eye. The trials were performed by a cohort consisting of one senior-surgeon and four junior-surgeons and manually annotated for bounding box locations of the cataract surgery tools (Figure 1) The surgical tools used included: forceps, diamond keratomes, viscoelastic cannulas, and cystotome needles. A FRCNN [3] was trained on a total of 130,614 frames for object detection. We used five-fold cross validation, using a leave-one-userout method. In this manner, all videos from one surgeon were reserved for testing and the frames from the remaining 20 videos were divided among training and validation. Network performance was evaluated via mean average precision (mAP), which is defined as the area under the precision/recall curve. Samples were considered correctly identified when the intersection over union (IoU) between the ground truth and predicted bounding boxes was greater than 0.5. Results: The overall mAP of the network was 0.63. Toolspecific mAPs ranged between 0.49 and 0.96 (Table 1). The high accuracy in detection of the cystotome needle is likely due to the distinct size and shape of the tool tip. The diamond keratome had the lowest mAP of any of the tools recognized, however this may be attributed to variations in the appearance of the tool tip (Figure 2). Conclusions: The FRCNN was able to recognize the surgical tools used in cataract surgery with reasonably high accuracy. Now that we know the network can sufficiently recognize the surgical tools, our next goal is to use this network to compute motion-based performance metrics. Future work seeks to validate these performance metrics against those obtained from sensor-based tracking and against expert evaluations. This serves as a first step towards providing consistent and accessible feedback for future trainees learning cataract surgery. </p>},
keywords = {},
pubstate = {published},
tppubtype = {conference}
}
March, L; Rodgers, JR; Jamzad, A; Santilli, AML; Hisey, Rebecca; McKay, D; Rudan, JF; Kaufmann, M; Ren, KYM; Fichtinger, G; Mousavi, P
Phase recognition and cautery localization in basal cell carcinoma surgical videos Journal Article
In: vol. 12034, pp. 508-517, 2022.
@article{fichtinger2022j,
title = {Phase recognition and cautery localization in basal cell carcinoma surgical videos},
author = {L March and JR Rodgers and A Jamzad and AML Santilli and Rebecca Hisey and D McKay and JF Rudan and M Kaufmann and KYM Ren and G Fichtinger and P Mousavi},
url = {https://www.spiedigitallibrary.org/conference-proceedings-of-spie/12034/1203427/Phase-recognition-and-cautery-localization-in-basal-cell-carcinoma-surgical/10.1117/12.2611837.short},
year = {2022},
date = {2022-01-01},
urldate = {2022-01-01},
volume = {12034},
pages = {508-517},
publisher = {SPIE},
abstract = {Surgical excision for basal cell carcinoma (BCC) is a common treatment to remove the affected areas of skin. Minimizing positive margins around excised tissue is essential for successful treatment. Residual cancer cells may result in repeat surgery; however, detecting remaining cancer can be challenging and time-consuming. Using chemical signal data acquired while tissue is excised with a cautery tool, the iKnife system can discriminate between healthy and cancerous tissue but lacks spatial information, making it difficult to navigate back to suspicious margins. Intraoperative videos of BCC excision allow cautery locations to be tracked, providing the sites of potential positive margins. We propose a deep learning approach using convolutional neural networks to recognize phases in the videos and subsequently track the cautery location, comparing two localization methods (supervised and semi-supervised …},
keywords = {},
pubstate = {published},
tppubtype = {article}
}
Barr, Colton; Hisey, Rebecca; Ungi, Tamas; Fichtinger, Gabor
Ultrasound Probe Pose Classification for Task Recognition in Central Venous Catheterization Conference
43rd Conference of the IEEE Engineering Medicine and Biology Society, 2021.
@conference{CBarr2021b,
title = {Ultrasound Probe Pose Classification for Task Recognition in Central Venous Catheterization},
author = {Colton Barr and Rebecca Hisey and Tamas Ungi and Gabor Fichtinger},
url = {https://labs.cs.queensu.ca/perklab/wp-content/uploads/sites/3/2024/02/CBarr2021a.pdf},
year = {2021},
date = {2021-10-01},
urldate = {2021-10-01},
booktitle = {43rd Conference of the IEEE Engineering Medicine and Biology Society},
abstract = {<p>Central Line Tutor is a system that facilitates real-time feedback during training for central venous catheterization. One limitation of Central Line Tutor is its reliance on expensive, cumbersome electromagnetic tracking to facilitate various training aids, including ultrasound task identification and segmentation of neck vasculature. The purpose of this study is to validate deep learning methods for vessel segmentation and ultrasound pose classification in order to mitigate the system’s reliance on electromagnetic tracking. A large dataset of segmented and classified ultrasound images was generated from participant data captured using Central Line Tutor. A U-Net architecture was used to perform vessel segmentation, while a shallow Convolutional Neural Network (CNN) architecture was designed to classify the pose of the ultrasound probe. A second classifier architecture was also tested that used the U-Net output as the CNN input. The mean testing set Intersect over Union score for U-Net cross-validation was 0.746 ± 0.052. The mean test set classification accuracy for the CNN was 92.0% ± 3.0, while the U-Net + CNN achieved 92.7% ± 2.1%. This study highlights the potential for deep learning on ultrasound images to replace the current electromagnetic tracking-based methods for vessel segmentation and ultrasound pose classification, and represents an important step towards removing the electromagnetic tracker altogether. Removing the need for an external tracking system would significantly reduce the cost of Central Line Tutor and make it far more accessible to the medical trainees that would benefit from it most.</p>},
keywords = {},
pubstate = {published},
tppubtype = {conference}
}
Hisey, Rebecca; Camire, Daenis; Erb, Jason; Howes, Daniel; Fichtinger, Gabor; Ungi, Tamas
System for central venous catheterization training using computer vision-based workflow feedback Journal Article
In: IEEE Transactions on Biomedical Engineering, 2021.
@article{Hisey2021b,
title = {System for central venous catheterization training using computer vision-based workflow feedback},
author = {Rebecca Hisey and Daenis Camire and Jason Erb and Daniel Howes and Gabor Fichtinger and Tamas Ungi},
year = {2021},
date = {2021-10-01},
urldate = {2021-10-01},
journal = {IEEE Transactions on Biomedical Engineering},
keywords = {},
pubstate = {published},
tppubtype = {article}
}
Barr, Colton; Hisey, Rebecca; Ungi, Tamas; Fichtinger, Gabor
Ultrasound Probe Pose Classification for Task Recognition in Central Venous Catheterization Conference
Imaging Network of Ontario Symposium, 2021.
@conference{CBarr2021a,
title = {Ultrasound Probe Pose Classification for Task Recognition in Central Venous Catheterization},
author = {Colton Barr and Rebecca Hisey and Tamas Ungi and Gabor Fichtinger},
year = {2021},
date = {2021-02-01},
urldate = {2021-02-01},
booktitle = {Imaging Network of Ontario Symposium},
keywords = {},
pubstate = {published},
tppubtype = {conference}
}
Hisey, Rebecca; Camire, Daenis; Erb, Jason; Howes, Daniel; Fichtinger, Gabor; Ungi, Tamas
Imaging Network of Ontario Symposium, 2021.
@conference{Hisey2021a,
title = {Central Line Tutor: using computer vision workflow recognition in a central venous catheterization training system},
author = {Rebecca Hisey and Daenis Camire and Jason Erb and Daniel Howes and Gabor Fichtinger and Tamas Ungi},
url = {https://labs.cs.queensu.ca/perklab/wp-content/uploads/sites/3/2024/02/RHisey_ImNO2021.pdf},
year = {2021},
date = {2021-02-01},
urldate = {2021-02-01},
booktitle = {Imaging Network of Ontario Symposium},
keywords = {},
pubstate = {published},
tppubtype = {conference}
}
O’Driscoll, Olivia; Hisey, Rebecca; Camire, Daenis; Erb, Jason; Howes, Daniel; Fichtinger, Gabor; Ungi, Tamas
SPIE Medical Imaging, 2021.
@conference{ODriscoll2021a,
title = {Object detection to compute performance metrics for skill assessment in central venous catheterization},
author = {Olivia O’Driscoll and Rebecca Hisey and Daenis Camire and Jason Erb and Daniel Howes and Gabor Fichtinger and Tamas Ungi},
url = {https://www.spiedigitallibrary.org/conference-proceedings-of-spie/11598/1159816/Object-detection-to-compute-performance-metrics-for-skill-assessment-in/10.1117/12.2581889.short?SSO=1
https://labs.cs.queensu.ca/perklab/wp-content/uploads/sites/3/2024/02/ODriscoll2021a.pdf},
year = {2021},
date = {2021-01-01},
urldate = {2021-01-01},
booktitle = {SPIE Medical Imaging},
keywords = {},
pubstate = {published},
tppubtype = {conference}
}
O’Driscoll, Olivia; Hisey, Rebecca; Camire, Daenis; Erb, Jason; Howes, Daniel; Fichtinger, Gabor; Ungi, Tamas
Imaging Network of Ontario Symposium, 2021.
@conference{ODriscoll2021b,
title = {Surgical tool tracking with object detection for performance assessment in central venous catheterization},
author = {Olivia O’Driscoll and Rebecca Hisey and Daenis Camire and Jason Erb and Daniel Howes and Gabor Fichtinger and Tamas Ungi},
url = {https://labs.cs.queensu.ca/perklab/wp-content/uploads/sites/3/2024/02/ODriscoll2021b.pdf},
year = {2021},
date = {2021-01-01},
urldate = {2021-01-01},
booktitle = {Imaging Network of Ontario Symposium},
keywords = {},
pubstate = {published},
tppubtype = {conference}
}
Barr, Colton; Hisey, Rebecca; Ungi, Tamas; Fichtinger, Gabor
Ultrasound probe pose classification for task recognition in central venous catheterization Journal Article
In: pp. 5023-5026, 2021.
@article{fichtinger2021l,
title = {Ultrasound probe pose classification for task recognition in central venous catheterization},
author = {Colton Barr and Rebecca Hisey and Tamas Ungi and Gabor Fichtinger},
url = {https://ieeexplore.ieee.org/abstract/document/9630033/},
year = {2021},
date = {2021-01-01},
pages = {5023-5026},
publisher = {IEEE},
abstract = {Central Line Tutor is a system that facilitates real-time feedback during training for central venous catheterization. One limitation of Central Line Tutor is its reliance on expensive, cumbersome electromagnetic tracking to facilitate various training aids, including ultrasound task identification and segmentation of neck vasculature. The purpose of this study is to validate deep learning methods for vessel segmentation and ultrasound pose classification in order to mitigate the system’s reliance on electromagnetic tracking. A large dataset of segmented and classified ultrasound images was generated from participant data captured using Central Line Tutor. A U-Net architecture was used to perform vessel segmentation, while a shallow Convolutional Neural Network (CNN) architecture was designed to classify the pose of the ultrasound probe. A second classifier architecture was also tested that used the U-Net output as …},
keywords = {},
pubstate = {published},
tppubtype = {article}
}
Hisey, Rebecca; Chen, Brian; Ungi, Tamas; Camire, Daenis; Erb, Jason; Howes, Daniel; Fichtinger, Gabor
Reinforcement learning approach for video-based task recognition in central venous catheterization Conference
Imaging Network of Ontario Symposium, 2020.
@conference{Hisey2020a,
title = {Reinforcement learning approach for video-based task recognition in central venous catheterization},
author = {Rebecca Hisey and Brian Chen and Tamas Ungi and Daenis Camire and Jason Erb and Daniel Howes and Gabor Fichtinger},
url = {https://labs.cs.queensu.ca/perklab/wp-content/uploads/sites/3/2024/02/RHisey_ImNO2020.pdf},
year = {2020},
date = {2020-06-01},
urldate = {2020-06-01},
booktitle = {Imaging Network of Ontario Symposium},
keywords = {},
pubstate = {published},
tppubtype = {conference}
}
Hisey, Rebecca; Chen, Brian; Camire, Daenis; Erb, Jason; Howes, Daniel; Fichtinger, Gabor; Ungi, Tamas
International Conference on Computer Assisted Radiology and Surgery, 2020.
@conference{Hisey2020b,
title = {Recognizing workflow tasks in central venous catheterization using convolutional neural networks and reinforcement learning},
author = {Rebecca Hisey and Brian Chen and Daenis Camire and Jason Erb and Daniel Howes and Gabor Fichtinger and Tamas Ungi},
url = {https://labs.cs.queensu.ca/perklab/wp-content/uploads/sites/3/2024/03/RHisey_CARS_2020_0.pdf},
year = {2020},
date = {2020-06-01},
urldate = {2020-06-01},
booktitle = {International Conference on Computer Assisted Radiology and Surgery},
pages = {94-95},
keywords = {},
pubstate = {published},
tppubtype = {conference}
}
Hisey, Rebecca; Ungi, Tamas; Camire, Daenis; Erb, Jason; Howes, Daniel; Fichtinger, Gabor
Comparison of convolutional neural networks for central venous catheterization tool detection Conference
Imaging Network of Ontario Symposium, Toronto, Ontario, 2019.
@conference{Hisey2019,
title = {Comparison of convolutional neural networks for central venous catheterization tool detection},
author = {Rebecca Hisey and Tamas Ungi and Daenis Camire and Jason Erb and Daniel Howes and Gabor Fichtinger},
url = {https://labs.cs.queensu.ca/perklab/wp-content/uploads/sites/3/2024/02/RHisey_ImNO2019_0.pdf},
year = {2019},
date = {2019-03-01},
urldate = {2019-03-01},
booktitle = {Imaging Network of Ontario Symposium},
address = {Toronto, Ontario},
keywords = {},
pubstate = {published},
tppubtype = {conference}
}
Isen, Jonah; Hisey, Rebecca; Ungi, Tamas; Fichtinger, Gabor
Retraining MobileNet with highly variable data for tool detection in central venous catheterization Conference
17th Annual Imaging Network Ontario Symposium (ImNO), Imaging Network Ontario (ImNO), London, Ontario, 2019.
@conference{Isen2019a,
title = {Retraining MobileNet with highly variable data for tool detection in central venous catheterization},
author = {Jonah Isen and Rebecca Hisey and Tamas Ungi and Gabor Fichtinger},
url = {https://labs.cs.queensu.ca/perklab/wp-content/uploads/sites/3/2024/02/Isen2019a.pdf},
year = {2019},
date = {2019-01-01},
urldate = {2019-01-01},
booktitle = {17th Annual Imaging Network Ontario Symposium (ImNO)},
publisher = {Imaging Network Ontario (ImNO)},
address = {London, Ontario},
keywords = {},
pubstate = {published},
tppubtype = {conference}
}
Isen, Jonah; Hisey, Rebecca; Ungi, Tamas; Fichtinger, Gabor
Utilizing a convolutional neural network for tool detection in central venous catheterization Conference
33rd International Congress & Exhibition on Computer Assisted Radiology and Surgery (CARS), Int J CARS, Rennes, France, 2019.
@conference{Isen2019b,
title = {Utilizing a convolutional neural network for tool detection in central venous catheterization},
author = {Jonah Isen and Rebecca Hisey and Tamas Ungi and Gabor Fichtinger},
url = {https://labs.cs.queensu.ca/perklab/wp-content/uploads/sites/3/2024/02/Isen2019b.pdf},
year = {2019},
date = {2019-01-01},
urldate = {2019-01-01},
booktitle = {33rd International Congress & Exhibition on Computer Assisted Radiology and Surgery (CARS)},
publisher = {Int J CARS},
address = {Rennes, France},
keywords = {},
pubstate = {published},
tppubtype = {conference}
}
Hisey, Rebecca; Ungi, Tamas; Holden, M.; Baum, Zachary M C; Keri, Zsuzsanna; McCallum, Caitlin; Howes, Daniel; Fichtinger, Gabor
Real-time workflow detection using webcam video for providing real-time feedback in central venous catheterization training Honorable Mention Conference
SPIE Medical Imaging 2018: Image-Guided Procedures, Robotic Interventions, and Modeling, 2018.
@conference{Hisey2018,
title = {Real-time workflow detection using webcam video for providing real-time feedback in central venous catheterization training},
author = {Rebecca Hisey and Tamas Ungi and M. Holden and Zachary M C Baum and Zsuzsanna Keri and Caitlin McCallum and Daniel Howes and Gabor Fichtinger},
url = {https://labs.cs.queensu.ca/perklab/wp-content/uploads/sites/3/2024/02/RHisey_SPIE2018_Full_02.pdf},
year = {2018},
date = {2018-01-01},
urldate = {2018-01-01},
booktitle = {SPIE Medical Imaging 2018: Image-Guided Procedures, Robotic Interventions, and Modeling},
keywords = {},
pubstate = {published},
tppubtype = {conference}
}
Hisey, Rebecca; Ungi, Tamas; Holden, M.; Baum, Zachary M C; Keri, Zsuzsanna; McCallum, Caitlin; Howes, Daniel; Fichtinger, Gabor
Imaging Network Ontario (IMNO), 2018.
@conference{Hisey2018b,
title = {Assessment of the use of webcam based workflow detection for providing real-time feedback in central venous catheterization training},
author = {Rebecca Hisey and Tamas Ungi and M. Holden and Zachary M C Baum and Zsuzsanna Keri and Caitlin McCallum and Daniel Howes and Gabor Fichtinger},
url = {https://labs.cs.queensu.ca/perklab/wp-content/uploads/sites/3/2024/02/Rebecca_ImNO2018_07.pdf},
year = {2018},
date = {2018-01-01},
urldate = {2018-01-01},
booktitle = {Imaging Network Ontario (IMNO)},
keywords = {},
pubstate = {published},
tppubtype = {conference}
}
Xia, Sean; Keri, Zsuzsanna; Holden, Matthew S; Hisey, Rebecca; Lia, Hillary; Ungi, Tamas; Mitchell, Christopher H; Fichtinger, Gabor
A learning curve analysis of ultrasound-guided in-plane and out-of-plane vascular access training with Perk Tutor Journal Article
In: vol. 10576, pp. 512-519, 2018.
@article{fichtinger2018i,
title = {A learning curve analysis of ultrasound-guided in-plane and out-of-plane vascular access training with Perk Tutor},
author = {Sean Xia and Zsuzsanna Keri and Matthew S Holden and Rebecca Hisey and Hillary Lia and Tamas Ungi and Christopher H Mitchell and Gabor Fichtinger},
url = {https://www.spiedigitallibrary.org/conference-proceedings-of-spie/10576/1057625/A-learning-curve-analysis-of-ultrasound-guided-in-plane-and/10.1117/12.2293789.short},
year = {2018},
date = {2018-01-01},
volume = {10576},
pages = {512-519},
publisher = {SPIE},
abstract = {PURPOSE
Under ultrasound guidance, procedures that have been traditionally performed using landmark approaches have become safer and more efficient. However, inexperienced trainees struggle with coordinating probe handling and needle insertion. We aimed to establish learning curves to identify the rate of acquisition of in-plane and out-of-plane vascular access skill in novice medical trainees.
METHODS
Thirty-eight novice participants were randomly assigned to perform either in-plane or out-of-plane insertions. Participants underwent baseline testing, four practice insertions (with 3D visualization assistance), and final testing; performance metrics were computed for all procedures. Five expert participants performed insertions in both approaches to establish expert performance metric benchmarks.
RESULTS In-plane novices (n=19) demonstrated significant final reductions in needle path inefficiency (45.8 …},
keywords = {},
pubstate = {published},
tppubtype = {article}
}
Under ultrasound guidance, procedures that have been traditionally performed using landmark approaches have become safer and more efficient. However, inexperienced trainees struggle with coordinating probe handling and needle insertion. We aimed to establish learning curves to identify the rate of acquisition of in-plane and out-of-plane vascular access skill in novice medical trainees.
METHODS
Thirty-eight novice participants were randomly assigned to perform either in-plane or out-of-plane insertions. Participants underwent baseline testing, four practice insertions (with 3D visualization assistance), and final testing; performance metrics were computed for all procedures. Five expert participants performed insertions in both approaches to establish expert performance metric benchmarks.
RESULTS In-plane novices (n=19) demonstrated significant final reductions in needle path inefficiency (45.8 …
Yang, Jianming; Hisey, Rebecca; Bierbrier, Joshua; Fichtinger, Gabor; Law, Christine; Holden, Matthew
Frame Selection Methods to Streamline Surgical Video Annotation for Tool Detection Tasks Proceedings Forthcoming
Forthcoming.
@proceedings{nokey,
title = {Frame Selection Methods to Streamline Surgical Video Annotation for Tool Detection Tasks},
author = {Jianming Yang and Rebecca Hisey and Joshua Bierbrier and Gabor Fichtinger and Christine Law and Matthew Holden},
abstract = {—Given the growing volume of surgical data and the
increasing demand for annotation, there is a pressing need to
streamline the annotation process for surgical videos. Previously,
annotation tools for object detection tasks have greatly evolved,
reducing time expense and enhancing ease. There are also many
initial frame selection approaches for Artificial Intelligence
(AI) assisted annotation tasks to further reduce human effort.
However, these methods have rarely been implemented and
reported in the context of surgical datasets, especially in cataract
surgery datasets. The identification of initial frames to annotate
before the use of any tools or algorithms determines annotation
efficiency. Therefore, in this paper, we chose to prioritize the
development of a method for selecting initial frames to facilitate
the subsequent automated annotation process. We propose a
customized initial frames selection method based on feature
clustering and compare it to commonly used temporal selection
methods. In each method, initial frames from cataract surgery
videos are selected to train a surgical tool detection model.
The model assists in the automated annotation process by
predicting bounding boxes for the surgery video objects in the
remaining frames. Evaluations of these methods are based on
how many edits users need to perform when annotating the
initial frames and how many edits users are expected to perform
to correct all predictions. Additionally, the total annotation cost
for each method is compared. Results indicate that on average,
the proposed cluster-based approach requires the fewest total
edits and exhibits the lowest total annotation cost compared
to conventional methods. These findings highlight a promising
direction for developing a complete application, featuring
streamlined AI-assisted annotation processes for surgical tool
detection tasks.},
keywords = {},
pubstate = {forthcoming},
tppubtype = {proceedings}
}
increasing demand for annotation, there is a pressing need to
streamline the annotation process for surgical videos. Previously,
annotation tools for object detection tasks have greatly evolved,
reducing time expense and enhancing ease. There are also many
initial frame selection approaches for Artificial Intelligence
(AI) assisted annotation tasks to further reduce human effort.
However, these methods have rarely been implemented and
reported in the context of surgical datasets, especially in cataract
surgery datasets. The identification of initial frames to annotate
before the use of any tools or algorithms determines annotation
efficiency. Therefore, in this paper, we chose to prioritize the
development of a method for selecting initial frames to facilitate
the subsequent automated annotation process. We propose a
customized initial frames selection method based on feature
clustering and compare it to commonly used temporal selection
methods. In each method, initial frames from cataract surgery
videos are selected to train a surgical tool detection model.
The model assists in the automated annotation process by
predicting bounding boxes for the surgery video objects in the
remaining frames. Evaluations of these methods are based on
how many edits users need to perform when annotating the
initial frames and how many edits users are expected to perform
to correct all predictions. Additionally, the total annotation cost
for each method is compared. Results indicate that on average,
the proposed cluster-based approach requires the fewest total
edits and exhibits the lowest total annotation cost compared
to conventional methods. These findings highlight a promising
direction for developing a complete application, featuring
streamlined AI-assisted annotation processes for surgical tool
detection tasks.
Yang, Jianming; Hisey, Rebecca; Bierbrier, Joshua; Fichtinger, Gabor; Law, Christine; Holden, Matthew
Frame Selection Methods to Streamline Surgical Video Annotation for Tool Detection Tasks Conference Forthcoming
IEEE, Forthcoming.
@conference{nokey,
title = {Frame Selection Methods to Streamline Surgical Video Annotation for Tool Detection Tasks},
author = {Jianming Yang and Rebecca Hisey and Joshua Bierbrier and Gabor Fichtinger and Christine Law and Matthew Holden},
publisher = {IEEE},
abstract = {Given the growing volume of surgical data and the
increasing demand for annotation, there is a pressing need to
streamline the annotation process for surgical videos. Previously,
annotation tools for object detection tasks have greatly evolved,
reducing time expense and enhancing ease. There are also many
initial frame selection approaches for Artificial Intelligence
(AI) assisted annotation tasks to further reduce human effort.
However, these methods have rarely been implemented and
reported in the context of surgical datasets, especially in cataract
surgery datasets. The identification of initial frames to annotate
before the use of any tools or algorithms determines annotation
efficiency. Therefore, in this paper, we chose to prioritize the
development of a method for selecting initial frames to facilitate
the subsequent automated annotation process. We propose a
customized initial frames selection method based on feature
clustering and compare it to commonly used temporal selection
methods. In each method, initial frames from cataract surgery
videos are selected to train a surgical tool detection model.
The model assists in the automated annotation process by
predicting bounding boxes for the surgery video objects in the
remaining frames. Evaluations of these methods are based on
how many edits users need to perform when annotating the
initial frames and how many edits users are expected to perform
to correct all predictions. Additionally, the total annotation cost
for each method is compared. Results indicate that on average,
the proposed cluster-based approach requires the fewest total
edits and exhibits the lowest total annotation cost compared
to conventional methods. These findings highlight a promising
direction for developing a complete application, featuring
streamlined AI-assisted annotation processes for surgical tool
detection tasks.},
keywords = {},
pubstate = {forthcoming},
tppubtype = {conference}
}
increasing demand for annotation, there is a pressing need to
streamline the annotation process for surgical videos. Previously,
annotation tools for object detection tasks have greatly evolved,
reducing time expense and enhancing ease. There are also many
initial frame selection approaches for Artificial Intelligence
(AI) assisted annotation tasks to further reduce human effort.
However, these methods have rarely been implemented and
reported in the context of surgical datasets, especially in cataract
surgery datasets. The identification of initial frames to annotate
before the use of any tools or algorithms determines annotation
efficiency. Therefore, in this paper, we chose to prioritize the
development of a method for selecting initial frames to facilitate
the subsequent automated annotation process. We propose a
customized initial frames selection method based on feature
clustering and compare it to commonly used temporal selection
methods. In each method, initial frames from cataract surgery
videos are selected to train a surgical tool detection model.
The model assists in the automated annotation process by
predicting bounding boxes for the surgery video objects in the
remaining frames. Evaluations of these methods are based on
how many edits users need to perform when annotating the
initial frames and how many edits users are expected to perform
to correct all predictions. Additionally, the total annotation cost
for each method is compared. Results indicate that on average,
the proposed cluster-based approach requires the fewest total
edits and exhibits the lowest total annotation cost compared
to conventional methods. These findings highlight a promising
direction for developing a complete application, featuring
streamlined AI-assisted annotation processes for surgical tool
detection tasks.