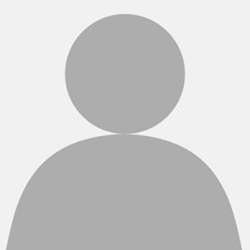
Victoria Wu
Victoria is a Perk Lab alumna, she was an undegraduate student in the Cognitive Science (COGS) Honor's program at the Queen's School of Computing. She curently works at Microsoft.
Ungi, Tamas; Greer, Hastings; Sunderland, Kyle R.; Wu, Victoria; Baum, Zachary M C; Schlenger, Christopher; Oetgen, Matthew; Cleary, Kevin; Aylward, Stephen; Fichtinger, Gabor
Automatic spine ultrasound segmentation for scoliosis visualization and measurement Journal Article
In: IEEE Transactions on Biomedical Engineering, vol. 67, no. 11, pp. 3234 - 3241, 2020.
@article{Ungi2020,
title = {Automatic spine ultrasound segmentation for scoliosis visualization and measurement},
author = {Tamas Ungi and Hastings Greer and Kyle R. Sunderland and Victoria Wu and Zachary M C Baum and Christopher Schlenger and Matthew Oetgen and Kevin Cleary and Stephen Aylward and Gabor Fichtinger},
url = {https://ieeexplore.ieee.org/document/9034149
https://labs.cs.queensu.ca/perklab/wp-content/uploads/sites/3/2024/02/Ungi2020.pdf},
doi = {10.1109/TBME.2020.2980540},
year = {2020},
date = {2020-03-01},
urldate = {2020-03-01},
journal = {IEEE Transactions on Biomedical Engineering},
volume = {67},
number = {11},
pages = {3234 - 3241},
abstract = {<p>\emph{Objective:} Integrate tracked ultrasound and AI methods to provide a safer and more accessible alternative to X-ray for scoliosis measurement. We propose automatic ultrasound segmentation for 3-dimensional spine visualization and scoliosis measurement to address difficulties in using ultrasound for spine imaging. \emph{Methods:} We trained a convolutional neural network for spine segmentation on ultrasound scans using data from eight healthy adult volunteers. We tested the trained network on eight pediatric patients. We evaluated image segmentation and 3-dimensional volume reconstruction for scoliosis measurement. \emph{Results:} As expected, fuzzy segmentation metrics reduced when trained networks were translated from healthy volunteers to patients. Recall decreased from 0.72 to 0.64 (8.2% decrease), and precision from 0.31 to 0.27 (3.7% decrease). However, after finding optimal thresholds for prediction maps, binary segmentation metrics performed better on patient data. Recall decreased from 0.98 to 0.97 (1.6% decrease), and precision from 0.10 to 0.06 (4.5% decrease). Segmentation prediction maps were reconstructed to 3-dimensional volumes and scoliosis was measured in all patients. Measurement in these reconstructions took less than 1 minute and had a maximum error of 2.2° compared to X-ray. \emph{Conclusion:} automatic spine segmentation makes scoliosis measurement both efficient and accurate in tracked ultrasound scans. \emph{Significance:} Automatic segmentation may overcome the limitations of tracked ultrasound that so far prevented its use as an alternative of X-ray in scoliosis measurement.</p>},
keywords = {},
pubstate = {published},
tppubtype = {article}
}
Wu, Victoria; Ungi, Tamas; Sunderland, Kyle R.; Pigeau, Grace; Schonewille, Abigael; Fichtinger, Gabor
SPIE Medical Imaging, 2020.
@conference{Wu2020a,
title = {Automatic segmentation of spinal ultrasound landmarks with U-net using multiple consecutive images for input},
author = {Victoria Wu and Tamas Ungi and Kyle R. Sunderland and Grace Pigeau and Abigael Schonewille and Gabor Fichtinger},
url = {https://labs.cs.queensu.ca/perklab/wp-content/uploads/sites/3/2024/02/CWu2020a-manuscript.pdf},
doi = {10.1117/12.2549584},
year = {2020},
date = {2020-01-01},
urldate = {2020-01-01},
booktitle = {SPIE Medical Imaging},
keywords = {},
pubstate = {published},
tppubtype = {conference}
}
Wu, Victoria; Ungi, Tamas; Sunderland, Kyle R.; Pigeau, Grace; Schonewille, Abigael; Fichtinger, Gabor
Using multiple frame U-net for automated segmentation of spinal ultrasound images Conference
18th Annual Imaging Network Ontario (ImNO) Symposium, 2020.
@conference{Wu2020b,
title = {Using multiple frame U-net for automated segmentation of spinal ultrasound images},
author = {Victoria Wu and Tamas Ungi and Kyle R. Sunderland and Grace Pigeau and Abigael Schonewille and Gabor Fichtinger},
url = {https://www.imno.ca/sites/default/files/ImNO2020Proceedings.pdf
https://labs.cs.queensu.ca/perklab/wp-content/uploads/sites/3/2024/02/Wu2020b.pdf},
year = {2020},
date = {2020-01-01},
urldate = {2020-01-01},
booktitle = {18th Annual Imaging Network Ontario (ImNO) Symposium},
keywords = {},
pubstate = {published},
tppubtype = {conference}
}
Pigeau, Grace; Elbatarny, Lydia; Wu, Victoria; Schonewille, Abigael; Fichtinger, Gabor; Ungi, Tamas
Ultrasound image simulation with generative adversarial network Journal Article
In: vol. 11315, pp. 54-60, 2020.
@article{fichtinger2020g,
title = {Ultrasound image simulation with generative adversarial network},
author = {Grace Pigeau and Lydia Elbatarny and Victoria Wu and Abigael Schonewille and Gabor Fichtinger and Tamas Ungi},
url = {https://www.spiedigitallibrary.org/conference-proceedings-of-spie/11315/1131508/Ultrasound-image-simulation-with-generative-adversarial-network/10.1117/12.2549592.short},
year = {2020},
date = {2020-01-01},
volume = {11315},
pages = {54-60},
publisher = {SPIE},
abstract = {PURPOSE
It is difficult to simulate realistic ultrasound images due to the complexity of acoustic artifacts that contribute to a real ultrasound image. We propose to evaluate the realism of ultrasound images simulated using a generative adversarial network.
METHODS
To achieve our goal, kidney ultrasounds were collected, and relevant anatomy was segmented to create anatomical label-maps using 3D Slicer. Adversarial networks were trained to generate ultrasound images from these labelmaps. Finally, a two-part survey of 4 participants with sonography experience was conducted to assess the realism of the generated images. The first part of the survey consisted of 50 kidney ultrasound images; half of which were real while the other half were simulated. Participants were asked to label each of the 50 ultrasound images as either real or simulated. In the second part of the survey, the participants were presented …},
keywords = {},
pubstate = {published},
tppubtype = {article}
}
It is difficult to simulate realistic ultrasound images due to the complexity of acoustic artifacts that contribute to a real ultrasound image. We propose to evaluate the realism of ultrasound images simulated using a generative adversarial network.
METHODS
To achieve our goal, kidney ultrasounds were collected, and relevant anatomy was segmented to create anatomical label-maps using 3D Slicer. Adversarial networks were trained to generate ultrasound images from these labelmaps. Finally, a two-part survey of 4 participants with sonography experience was conducted to assess the realism of the generated images. The first part of the survey consisted of 50 kidney ultrasound images; half of which were real while the other half were simulated. Participants were asked to label each of the 50 ultrasound images as either real or simulated. In the second part of the survey, the participants were presented …
Gerolami, Justin; Wu, Victoria; Fauerbach, P Nasute; Jabs, Doris; Engel, Cecil Jay; Rudan, J; Merchant, Shaila; Walker, Ross; Anas, Emran Mohammad Abu; Abolmaesumi, Purang; Fichtinger, Gabor; Ungi, Tamas; Mousavi, Parvin
An End-to-End Solution for Automatic Contouring of Tumor Region in Intraoperative Images of Breast Lumpectomy Journal Article
In: pp. 2003-2006, 2020.
@article{fichtinger2020w,
title = {An End-to-End Solution for Automatic Contouring of Tumor Region in Intraoperative Images of Breast Lumpectomy},
author = {Justin Gerolami and Victoria Wu and P Nasute Fauerbach and Doris Jabs and Cecil Jay Engel and J Rudan and Shaila Merchant and Ross Walker and Emran Mohammad Abu Anas and Purang Abolmaesumi and Gabor Fichtinger and Tamas Ungi and Parvin Mousavi},
url = {https://ieeexplore.ieee.org/abstract/document/9176505/},
year = {2020},
date = {2020-01-01},
pages = {2003-2006},
publisher = {IEEE},
abstract = {Breast-conserving surgery, also known as lumpectomy, is an early stage breast cancer treatment that aims to spare as much healthy breast tissue as possible. A risk associated with lumpectomy is the presence of cancer positive margins post operation. Surgical navigation has been shown to reduce cancer positive margins but requires manual segmentation of the tumor intraoperatively. In this paper, we propose an end-to-end solution for automatic contouring of breast tumor from intraoperative ultrasound images using two convolutional neural network architectures, the U-Net and residual U-Net. The networks are trained on annotated intraoperative breast ultrasound images and evaluated on the quality of predicted segmentations. This work brings us one step closer to providing surgeons with an automated surgical navigation system that helps reduce cancer-positive margins during lumpectomy.},
keywords = {},
pubstate = {published},
tppubtype = {article}
}
Wu, Victoria; Asselin, Mark; Ungi, Tamas; Fichtinger, Gabor
Detection of Spinal Ultrasound Landmarks Using Convolutional Neural Networks Conference
33rd International Congress & Exhibition on Computer Assisted Radiology and Surgery (CARS), vol. 14, Int J CARS, Rennes, France, 2019.
@conference{Wu2019b,
title = {Detection of Spinal Ultrasound Landmarks Using Convolutional Neural Networks},
author = {Victoria Wu and Mark Asselin and Tamas Ungi and Gabor Fichtinger},
url = {https://labs.cs.queensu.ca/perklab/wp-content/uploads/sites/3/2024/02/Wu2019b.pdf},
doi = {https://doi.org/10.1007/s11548-019-01969-3},
year = {2019},
date = {2019-01-01},
urldate = {2019-01-01},
booktitle = {33rd International Congress & Exhibition on Computer Assisted Radiology and Surgery (CARS)},
volume = {14},
publisher = {Int J CARS},
address = {Rennes, France},
keywords = {},
pubstate = {published},
tppubtype = {conference}
}
Wu, Victoria; Ungi, Tamas; Fichtinger, Gabor
Using Deep Learning for Transverse Process Detection in Spinal Ultrasounds Conference
17th Annual Imaging Network Ontario Symposium (ImNO), Imaging Network Ontario (ImNO), London, Ontario, 2019.
@conference{Wu2019a,
title = {Using Deep Learning for Transverse Process Detection in Spinal Ultrasounds},
author = {Victoria Wu and Tamas Ungi and Gabor Fichtinger},
url = {https://labs.cs.queensu.ca/perklab/wp-content/uploads/sites/3/2024/02/Wu2019a.pdf},
year = {2019},
date = {2019-01-01},
urldate = {2019-01-01},
booktitle = {17th Annual Imaging Network Ontario Symposium (ImNO)},
publisher = {Imaging Network Ontario (ImNO)},
address = {London, Ontario},
keywords = {},
pubstate = {published},
tppubtype = {conference}
}