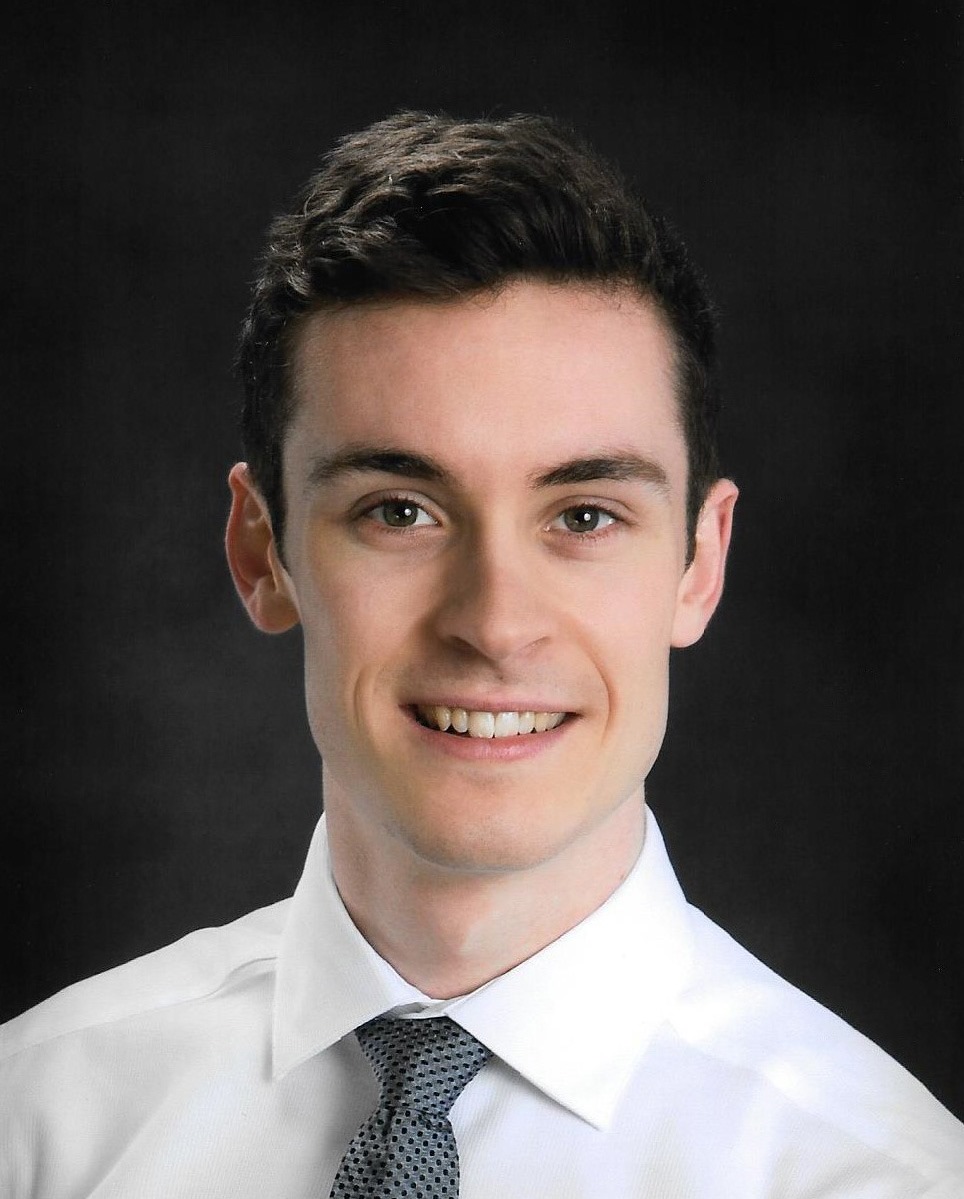
Biography
Colton Barr is an MD / PhD student in the School of Computing at Queen’s University supervised by Professor Gabor Fichtinger and Professor Parvin Mousavi. He is currently completing his second internship as a visiting researcher at the Golby Lab at Brigham and Women’s Hospital under the supervision of Dr. Alexandra Golby. His research interests lie at the intersection of surgical navigation, open-source software development and artificial intelligence, with a particular focus on building accessible solutions for deployment in limited-resource healthcare settings.
Colton completed his BCompH at Queen’s in Biomedical Computing in 2019 and his MSc in Computing in 2022 at the Perk Lab.
Publications
Elkind, Emese; Barr, Keiran; Barr, Colton; Moga, Kristof; Garamvolgy, Tivadar; Haidegger, Tamas; Ungi, Tamas; Fichtinger, Gabor
Modifying Radix Lenses to Survive Low-Cost Sterilization: An Exploratory Study Conference
Imaging Network of Ontario (ImNO) Symposium, Imaging Network of Ontario (ImNO) Symposium, 2024.
@conference{Elkind2024,
title = {Modifying Radix Lenses to Survive Low-Cost Sterilization: An Exploratory Study},
author = {Emese Elkind and Keiran Barr and Colton Barr and Kristof Moga and Tivadar Garamvolgy and Tamas Haidegger and Tamas Ungi and Gabor Fichtinger},
url = {https://labs.cs.queensu.ca/perklab/wp-content/uploads/sites/3/2024/10/EmeseElkindImNO2024-2.docx},
year = {2024},
date = {2024-03-19},
urldate = {2024-03-19},
booktitle = {Imaging Network of Ontario (ImNO) Symposium},
publisher = {Imaging Network of Ontario (ImNO) Symposium},
abstract = {INTRODUCTION: A major challenge with deploying infrared camera-tracked surgical navigation solutions, such as NousNav [1], in low-resource settings is the high cost and unavailability of disposable retroreflective infrared markers. Developing an accessible method to reuse and sterilize retroreflective markers could lead to significant increase in the uptake of this technology. As none of the known infrared markers can endure standard autoclaving and most places do not have access to gas sterilization, attention is focused on cold liquid sterilisation methods commonly used in laparoscopy and other optical tools that cannot be sterilized in a conventional autoclave.
METHODS: We propose to modify NDI Radix™ Lens [1], single-use retroreflective spherical marker manufactured by Northern Digital, Waterloo, Canada. Radix lenses are uniquely promising candidates for liquid sterilization given their smooth, spherical surface. This quality also makes them easier to clean perioperatively compared to other retroreflective infrared marker designs. Initial experiments show that liquid sterilization agents degrade the marker’s retroreflective gold coating (Fig. 1). Hence the objective of this project is to develop a method to protect the Radix Lenses with a layer of coating material that does not allow the sanitizing agent to degrade the coating to enable the lens to survive multiple sanitation cycles while retaining sufficient tracking accuracy. We employed two cold liquid sterilisation agents, household bleach which is a common ingredient of liquid sterilisation solutions and Sekusept™ Aktiv (Ecolab, Saint Paul, MN, USA), which is widely known for sterilizing laparoscopy instruments. Store-bought nail polish and Zink-Alu Spray were used to coat the lenses. Data were obtained by recording five tests each with five rounds of sterilization, each tested with six trials, for a total of 150 recordings. The five tests were as follows: 1) Radix lens coated with nail polish and bleached, 2) uncoated and bleached, 3) coated with nail polish and sanitised, 4) uncoated and sanitised, and 5) coated with Zink-Alu Spray and sanitised. To assess the impact of the sterilization on the lens’s fiducial localization error, two metal marker frames equipped with four sockets designed for the Radix lenses were used. The reference marker frame was secured to a flat table while the other marker frame moved along a fixed path on the table. The position and orientation of the marker clusters were streamed into 3D Slicer using the Public Library for Ultrasound Toolkit (PLUS). A plane was then fit to the recorded marker poses in 3D Slicer using Iterative Closest Point and the marker registration error was computed. Distance from the camera, angle of view, and distance from the edges of the field of view were held constant.
RESULTS: With each round of sterilization, the error of coated lenses was lower than the unprotected lenses, and the error showed a slightly increasing trend (Fig. 2). The lenses appeared fainter in the tracking software the lenses appeared fainter while all lenses remained trackable and visible despite the significant removal of reflective coating.
When reflective coating was fully rubbed off the lenses, the tracking software could still localize the markers; however, the lenses did appear much fainter in the tracking software. We observed that the reflective coating rubs off the lens in routine handling, and recoating with Zink-Alu spray can partially restore marker visibility. Using protective nail polish coating prevented the reflective coating from rubbing off altogether.
CONCLUSIONS: This exploratory study represents a promising step toward achieving low-cost sterilization of retroreflective infrared markers. Studies with the NousNav system need to be undertaken to measure the extent of degradation in tracking accuracy is tolerable as a side effect of marker sterilization. Before using coated Radix lenses on human subjects, it must be verified that the protective coating (common nail polish in our study) is fully biocompatible and remains undamaged by the cold sterilization agent (Sekusept™ Aktiv in our study.)
REFERENCES: [1] NousNav: A low-cost neuronavigation system for deployment in lower-resource settings, International Journal of Computer Assisted Radiology and Surgery, 2022 Sep;17(9):1745-1750. [2] NDI Radix™ Lens (https://www.ndigital.com/optical-measurement-technology/radix-lens/) },
keywords = {},
pubstate = {published},
tppubtype = {conference}
}
METHODS: We propose to modify NDI Radix™ Lens [1], single-use retroreflective spherical marker manufactured by Northern Digital, Waterloo, Canada. Radix lenses are uniquely promising candidates for liquid sterilization given their smooth, spherical surface. This quality also makes them easier to clean perioperatively compared to other retroreflective infrared marker designs. Initial experiments show that liquid sterilization agents degrade the marker’s retroreflective gold coating (Fig. 1). Hence the objective of this project is to develop a method to protect the Radix Lenses with a layer of coating material that does not allow the sanitizing agent to degrade the coating to enable the lens to survive multiple sanitation cycles while retaining sufficient tracking accuracy. We employed two cold liquid sterilisation agents, household bleach which is a common ingredient of liquid sterilisation solutions and Sekusept™ Aktiv (Ecolab, Saint Paul, MN, USA), which is widely known for sterilizing laparoscopy instruments. Store-bought nail polish and Zink-Alu Spray were used to coat the lenses. Data were obtained by recording five tests each with five rounds of sterilization, each tested with six trials, for a total of 150 recordings. The five tests were as follows: 1) Radix lens coated with nail polish and bleached, 2) uncoated and bleached, 3) coated with nail polish and sanitised, 4) uncoated and sanitised, and 5) coated with Zink-Alu Spray and sanitised. To assess the impact of the sterilization on the lens’s fiducial localization error, two metal marker frames equipped with four sockets designed for the Radix lenses were used. The reference marker frame was secured to a flat table while the other marker frame moved along a fixed path on the table. The position and orientation of the marker clusters were streamed into 3D Slicer using the Public Library for Ultrasound Toolkit (PLUS). A plane was then fit to the recorded marker poses in 3D Slicer using Iterative Closest Point and the marker registration error was computed. Distance from the camera, angle of view, and distance from the edges of the field of view were held constant.
RESULTS: With each round of sterilization, the error of coated lenses was lower than the unprotected lenses, and the error showed a slightly increasing trend (Fig. 2). The lenses appeared fainter in the tracking software the lenses appeared fainter while all lenses remained trackable and visible despite the significant removal of reflective coating.
When reflective coating was fully rubbed off the lenses, the tracking software could still localize the markers; however, the lenses did appear much fainter in the tracking software. We observed that the reflective coating rubs off the lens in routine handling, and recoating with Zink-Alu spray can partially restore marker visibility. Using protective nail polish coating prevented the reflective coating from rubbing off altogether.
CONCLUSIONS: This exploratory study represents a promising step toward achieving low-cost sterilization of retroreflective infrared markers. Studies with the NousNav system need to be undertaken to measure the extent of degradation in tracking accuracy is tolerable as a side effect of marker sterilization. Before using coated Radix lenses on human subjects, it must be verified that the protective coating (common nail polish in our study) is fully biocompatible and remains undamaged by the cold sterilization agent (Sekusept™ Aktiv in our study.)
REFERENCES: [1] NousNav: A low-cost neuronavigation system for deployment in lower-resource settings, International Journal of Computer Assisted Radiology and Surgery, 2022 Sep;17(9):1745-1750. [2] NDI Radix™ Lens (https://www.ndigital.com/optical-measurement-technology/radix-lens/)
Barr, Colton; Groves, Leah; Ungi, Tamas; Siemens, D Robert; Diao, Babacar; Kikinis, Ron; Mousavi, Parvin; Fichtinger, Gabor
Extracting 3D Prostate Geometry from 2D Optically-Tracked Transrectal Ultrasound Images Journal Article
In: pp. 32-37, 2024.
@article{barr2024,
title = {Extracting 3D Prostate Geometry from 2D Optically-Tracked Transrectal Ultrasound Images},
author = {Colton Barr and Leah Groves and Tamas Ungi and D Robert Siemens and Babacar Diao and Ron Kikinis and Parvin Mousavi and Gabor Fichtinger},
year = {2024},
date = {2024-01-01},
pages = {32-37},
publisher = {IEEE},
abstract = {The technical challenges of traditional transrectal ultrasound-guided prostate biopsy, combined with the limited availability of more advanced prostate imaging techniques, have exacerbated existing differences in prostate cancer outcomes between high-resource and low-resource healthcare settings. The objective of this paper is to improve the tools available to clinicians in low-resource settings by working towards an inexpensive ultrasound-guided prostate biopsy navigation system. The principal contributions detailed here are the design, implementation, and testing of a system capable of generating a 3D model of the prostate from spatially-tracked 2D ultrasound images. The system uses open-source software, low-cost materials, and deep learning to segment and localize cross-sections of the prostate in order to produce a patient-specific 3D prostate model. A user study was performed to evaluate the …},
keywords = {},
pubstate = {published},
tppubtype = {article}
}
Orosz, Gábor; Szabó, Róbert Zsolt; Ungi, Tamás; Barr, Colton; Yeung, Chris; Fichtinger, Gábor; Gál, János; Haidegger, Tamás
Lung Ultrasound Imaging and Image Processing with Artificial Intelligence Methods for Bedside Diagnostic Examinations Journal Article
In: Acta Polytechnica Hungarica, vol. 20, iss. 8, 2023.
@article{fichtinger2023d,
title = {Lung Ultrasound Imaging and Image Processing with Artificial Intelligence Methods for Bedside Diagnostic Examinations},
author = {Gábor Orosz and Róbert Zsolt Szabó and Tamás Ungi and Colton Barr and Chris Yeung and Gábor Fichtinger and János Gál and Tamás Haidegger},
url = {https://acta.uni-obuda.hu/Orosz_Szabo_Ungi_Barr_Yeung_Fichtinger_Gal_Haidegger_137.pdf},
year = {2023},
date = {2023-01-01},
journal = {Acta Polytechnica Hungarica},
volume = {20},
issue = {8},
abstract = {Artificial Intelligence-assisted radiology has shown to offer significant benefits in clinical care. Physicians often face challenges in identifying the underlying causes of acute respiratory failure. One method employed by experts is the utilization of bedside lung ultrasound, although it has a significant learning curve. In our study, we explore the potential of a Machine Learning-based automated decision-support system to assist inexperienced practitioners in interpreting lung ultrasound scans. This system incorporates medical ultrasound, advanced data processing techniques, and a neural network implementation to achieve its objective. The article provides a comprehensive overview of the steps involved in data preparation and the implementation of the neural network. The accuracy and error rate of the most effective model are presented, accompanied by illustrative examples of their predictions. Furthermore, the paper concludes with an evaluation of the results, identification of limitations, and recommendations for future enhancements.},
keywords = {},
pubstate = {published},
tppubtype = {article}
}
Szabó, Róbert Zsolt; Orosz, Gábor; Ungi, Tamás; Barr, Colton; Yeung, Chris; Incze, Roland; Fichtinger, Gabor; Gál, János; Haidegger, Tamás
Automation of lung ultrasound imaging and image processing for bedside diagnostic examinations Journal Article
In: pp. 000779-000784, 2023.
@article{fichtinger2023h,
title = {Automation of lung ultrasound imaging and image processing for bedside diagnostic examinations},
author = {Róbert Zsolt Szabó and Gábor Orosz and Tamás Ungi and Colton Barr and Chris Yeung and Roland Incze and Gabor Fichtinger and János Gál and Tamás Haidegger},
url = {https://ieeexplore.ieee.org/abstract/document/10158672/},
year = {2023},
date = {2023-01-01},
pages = {000779-000784},
publisher = {IEEE},
abstract = {The causes of acute respiratory failure can be difficult to identify for physicians. Experts can differentiate these causes using bedside lung ultrasound, but lung ultrasound has a considerable learning curve. We investigate if an automated decision-support system could help novices interpret lung ultrasound scans. The system utilizes medical ultrasound, data processing, and a neural network implementation to achieve this goal. The article details the steps taken in the data preparation, and the implementation of the neural network. The best model’s accuracy and error rate are presented, along with examples of its predictions. The paper concludes with an evaluation of the results, identification of limitations, and suggestions for future improvements.},
keywords = {},
pubstate = {published},
tppubtype = {article}
}
Barr, Colton; Hisey, Rebecca; Ungi, Tamas; Fichtinger, Gabor
Ultrasound Probe Pose Classification for Task Recognition in Central Venous Catheterization Conference
43rd Conference of the IEEE Engineering Medicine and Biology Society, 2021.
@conference{CBarr2021b,
title = {Ultrasound Probe Pose Classification for Task Recognition in Central Venous Catheterization},
author = {Colton Barr and Rebecca Hisey and Tamas Ungi and Gabor Fichtinger},
url = {https://labs.cs.queensu.ca/perklab/wp-content/uploads/sites/3/2024/02/CBarr2021a.pdf},
year = {2021},
date = {2021-10-01},
urldate = {2021-10-01},
booktitle = {43rd Conference of the IEEE Engineering Medicine and Biology Society},
abstract = {<p>Central Line Tutor is a system that facilitates real-time feedback during training for central venous catheterization. One limitation of Central Line Tutor is its reliance on expensive, cumbersome electromagnetic tracking to facilitate various training aids, including ultrasound task identification and segmentation of neck vasculature. The purpose of this study is to validate deep learning methods for vessel segmentation and ultrasound pose classification in order to mitigate the system’s reliance on electromagnetic tracking. A large dataset of segmented and classified ultrasound images was generated from participant data captured using Central Line Tutor. A U-Net architecture was used to perform vessel segmentation, while a shallow Convolutional Neural Network (CNN) architecture was designed to classify the pose of the ultrasound probe. A second classifier architecture was also tested that used the U-Net output as the CNN input. The mean testing set Intersect over Union score for U-Net cross-validation was 0.746 ± 0.052. The mean test set classification accuracy for the CNN was 92.0% ± 3.0, while the U-Net + CNN achieved 92.7% ± 2.1%. This study highlights the potential for deep learning on ultrasound images to replace the current electromagnetic tracking-based methods for vessel segmentation and ultrasound pose classification, and represents an important step towards removing the electromagnetic tracker altogether. Removing the need for an external tracking system would significantly reduce the cost of Central Line Tutor and make it far more accessible to the medical trainees that would benefit from it most.</p>},
keywords = {},
pubstate = {published},
tppubtype = {conference}
}
Barr, Colton; Hisey, Rebecca; Ungi, Tamas; Fichtinger, Gabor
Ultrasound Probe Pose Classification for Task Recognition in Central Venous Catheterization Conference
Imaging Network of Ontario Symposium, 2021.
@conference{CBarr2021a,
title = {Ultrasound Probe Pose Classification for Task Recognition in Central Venous Catheterization},
author = {Colton Barr and Rebecca Hisey and Tamas Ungi and Gabor Fichtinger},
year = {2021},
date = {2021-02-01},
urldate = {2021-02-01},
booktitle = {Imaging Network of Ontario Symposium},
keywords = {},
pubstate = {published},
tppubtype = {conference}
}
Barr, Colton; Hisey, Rebecca; Ungi, Tamas; Fichtinger, Gabor
Ultrasound probe pose classification for task recognition in central venous catheterization Journal Article
In: pp. 5023-5026, 2021.
@article{fichtinger2021l,
title = {Ultrasound probe pose classification for task recognition in central venous catheterization},
author = {Colton Barr and Rebecca Hisey and Tamas Ungi and Gabor Fichtinger},
url = {https://ieeexplore.ieee.org/abstract/document/9630033/},
year = {2021},
date = {2021-01-01},
pages = {5023-5026},
publisher = {IEEE},
abstract = {Central Line Tutor is a system that facilitates real-time feedback during training for central venous catheterization. One limitation of Central Line Tutor is its reliance on expensive, cumbersome electromagnetic tracking to facilitate various training aids, including ultrasound task identification and segmentation of neck vasculature. The purpose of this study is to validate deep learning methods for vessel segmentation and ultrasound pose classification in order to mitigate the system’s reliance on electromagnetic tracking. A large dataset of segmented and classified ultrasound images was generated from participant data captured using Central Line Tutor. A U-Net architecture was used to perform vessel segmentation, while a shallow Convolutional Neural Network (CNN) architecture was designed to classify the pose of the ultrasound probe. A second classifier architecture was also tested that used the U-Net output as …},
keywords = {},
pubstate = {published},
tppubtype = {article}
}
Barr, Colton; Lasso, Andras; Asselin, Mark; Pieper, Steve; Robertson, Faith C.; Gormley, William B.; Fichtinger, Gabor
Medical Imaging 2020: Image-Guided Procedures, Robotic Interventions and Modeling, vol. 11315, SPIE SPIE, Houston, Texas, United States, 2020.
@conference{BarrC2020,
title = {Towards portable image guidance and automatic patient registration using an RGB-D camera and video projector},
author = {Colton Barr and Andras Lasso and Mark Asselin and Steve Pieper and Faith C. Robertson and William B. Gormley and Gabor Fichtinger},
url = {https://labs.cs.queensu.ca/perklab/wp-content/uploads/sites/3/2024/02/Barr2020.pdf},
doi = {10.1117/12.2549723},
year = {2020},
date = {2020-01-01},
urldate = {2020-01-01},
booktitle = {Medical Imaging 2020: Image-Guided Procedures, Robotic Interventions and Modeling},
volume = {11315},
publisher = {SPIE},
address = {Houston, Texas, United States},
organization = {SPIE},
keywords = {},
pubstate = {published},
tppubtype = {conference}
}
Barr, Colton
SlicerChat: Building a Local Chatbot for 3D Slicer Unpublished Forthcoming
Forthcoming.
@unpublished{nokey,
title = {SlicerChat: Building a Local Chatbot for 3D Slicer},
author = {Colton Barr},
url = {https://labs.cs.queensu.ca/perklab/wp-content/uploads/sites/3/2024/06/SlicerChat_2024.pdf},
keywords = {},
pubstate = {forthcoming},
tppubtype = {unpublished}
}