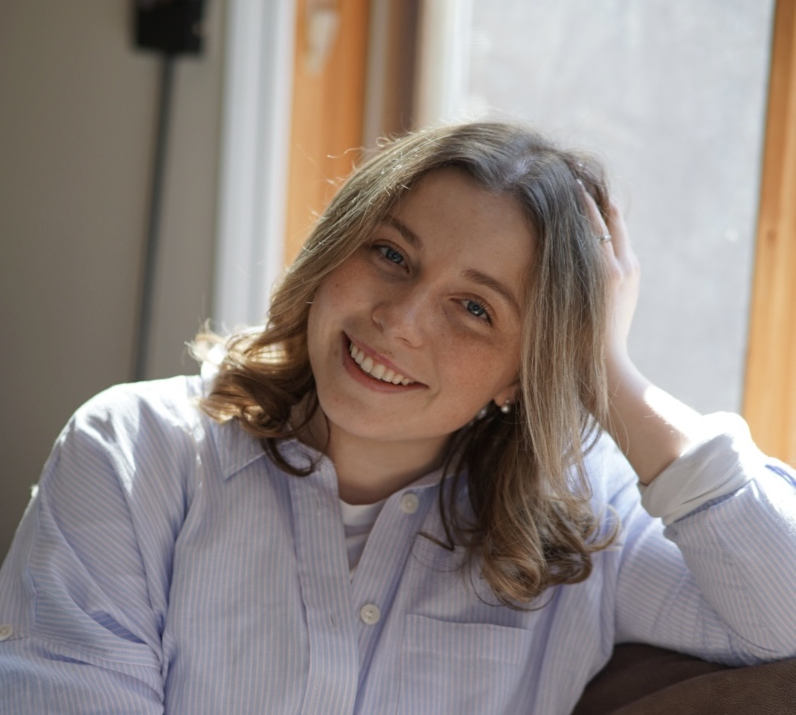
Laura Connolly
Biography
Laura Connolly is a Ph.D. Candidate in Electrical Engineering at Queen’s University in Kingston, ON, Canada. She is mentored by Dr. Gabor Fichtinger, Dr. Parvin Mousavi and Dr. Russell H. Taylor. She has recently completed her second visiting studentship at Johns Hopkins University in Baltimore, MD, USA in the Laboratory for Computational Sensing and Robotics (LCSR).
Laura began researching computer-integrated surgery in 2018 as an undergraduate researcher before entering her graduate studies in 2020. Her research is now primarily focused on the application of robotics and image guidance for margin detection in breast-conserving surgery.
Publications
Elkind, Emese; Tun, Aung Tin; Radcliffe, Olivia; Connolly, Laura; Davison, Colleen; Purkey, Eva; Mousavi, Parvin; Fichtinger, Gabor; Thornton, Kanchana
INOVAIT Image-Guided Therapy (IGT) x Imaging Network Ontario (ImNO), 2025.
@conference{Elkind2025b,
title = {Developing low-cost 3D-printed prosthetics with a functional wrist for patients along the Thai-Myanmar border},
author = {Emese Elkind and Aung Tin Tun and Olivia Radcliffe and Laura Connolly and Colleen Davison and Eva Purkey and Parvin Mousavi and Gabor Fichtinger and Kanchana Thornton
},
url = {https://labs.cs.queensu.ca/perklab/eelkind_imno2025_poster_wrist/},
year = {2025},
date = {2025-03-05},
urldate = {2025-03-05},
publisher = {INOVAIT Image-Guided Therapy (IGT) x Imaging Network Ontario (ImNO)},
abstract = {INTRODUCTION: Inadequacies in the Burmese healthcare system, heightened by the 2021 military coup and related civil war in Myanmar and the COVID-19 pandemic, have contributed to an influx of refugees to Thailand to seek medical aid. An estimated 1.5 million Myanmar nationals entered Thailand since January 2023 [5]. Without immigration status, these refugees are unable to receive healthcare. Burma Children Medical Fund (BCMF) is a nonprofit based in Mae Sot, Tak, Thailand that focuses on funding underserved Burmese communities’ medical treatment and providing support services, including accessible prosthetics for refugees who have experienced limb loss [1]. Prosthetics in lower-income countries are usually passive, meaning they lack mechanisms to restore critical limb functions such as gripping, rotation, or complex hand movements. Therefore, patients cannot fully perform their daily functions, impacting their abilities to work and affecting family caretakers. BCMF aims to make prosthetics that work best in low-resource settings using open-source designs, which only allow for fixed hand positions. The usage of prosthetic arms depends heavily on their functionality and comfort. Patients are more likely to consistently use prosthetics if it aids them in returning to normalcy. In this study, we present a design for an interchangeable and functional prosthetic wrist that enables critical hand motions such as rotation.
METHODS: BCMF currently provides custom-fitted, low-cost, 3D-printed prostheses that are found on Thingiverse, a public library of 3D designs. One such design is the Kwawu Arm 2.0 [2], which can be adjusted with OpenSCAD [4], a software for modifying 3D CAD models to fit the recipient's measurements. To maintain BCMF’s workflow, the interchangeable wrist model was created using the 3D design software, Autodesk Fusion 360, and designs from open sourced Quick-Connect Wrist designs found on Thingiverse [3]. The wrist was merged onto the Kwawu Arm, printed, assembled, and tested for durability and comfort both with and without patients. This is an iterative process where patient feedback ensures the prosthetics cater to the diverse needs of the recipients.
RESULTS: Since the launch of the prosthetics project in 2019, BCMF has provided 3D-printed prosthetics to 76 patients. The interchangeable hand provides a solution to many patients' everyday activities and can rotate the hand 360 degrees (Fig.2) and has been tested on and used by one patient thus far (Fig.1).
CONCLUSIONS: The BCMF prosthetics project provides a low-cost solution to healthcare challenges in the context of poly-crisis experienced in Myanmar, enhancing the resilience and adaptability of affected refugee communities. The collaboration between BCMF and Queen’s University demonstrates the potential for future partnerships between educational institutions and NGOs to address health care access disparities. Future work includes continuing to fill the gap between open-sourced models and patient-specific needs to refine the 3D-printing workflow by continuing to create customizable, generalized designs. We also plan to test the interchangeable wrist with more patients and develop body-powered prosthetic designs to support more critical movements.
REFERENCES: [1]Burma Children Medical Fund - Mae Sot, Thailand. BCMF | Burma Children Medical Fund - Mae Sot, Thailand - Operating to give people a future. (n.d.). https://burmachildren.com/ [2]Buchanan, J. (2018, March 27). Kwawu Arm 2.0 - Prosthetic - socket version. Thingiverse. https://www.thingiverse.com/thing:2841281 [3]NIOP. (2022, February 9). NIOP Q-C V1 quick-connect wrist. Thingiverse. http://www.thingiverse.com/thing:5238794 [4]OpenSCAD. The Programmers Solid 3D CAD Modeller. (n.d.). https://openscad.org/ [5]UN. Overview of Myanmar nationals in Thailand. IOM UN migration. https://thailand.iom.int/sites/g/files/tmzbdl1371/files/documents/2024-10/overview-of-myanmar-nationals-in-thailand-october-24.pdf
},
keywords = {},
pubstate = {published},
tppubtype = {conference}
}
METHODS: BCMF currently provides custom-fitted, low-cost, 3D-printed prostheses that are found on Thingiverse, a public library of 3D designs. One such design is the Kwawu Arm 2.0 [2], which can be adjusted with OpenSCAD [4], a software for modifying 3D CAD models to fit the recipient's measurements. To maintain BCMF’s workflow, the interchangeable wrist model was created using the 3D design software, Autodesk Fusion 360, and designs from open sourced Quick-Connect Wrist designs found on Thingiverse [3]. The wrist was merged onto the Kwawu Arm, printed, assembled, and tested for durability and comfort both with and without patients. This is an iterative process where patient feedback ensures the prosthetics cater to the diverse needs of the recipients.
RESULTS: Since the launch of the prosthetics project in 2019, BCMF has provided 3D-printed prosthetics to 76 patients. The interchangeable hand provides a solution to many patients' everyday activities and can rotate the hand 360 degrees (Fig.2) and has been tested on and used by one patient thus far (Fig.1).
CONCLUSIONS: The BCMF prosthetics project provides a low-cost solution to healthcare challenges in the context of poly-crisis experienced in Myanmar, enhancing the resilience and adaptability of affected refugee communities. The collaboration between BCMF and Queen’s University demonstrates the potential for future partnerships between educational institutions and NGOs to address health care access disparities. Future work includes continuing to fill the gap between open-sourced models and patient-specific needs to refine the 3D-printing workflow by continuing to create customizable, generalized designs. We also plan to test the interchangeable wrist with more patients and develop body-powered prosthetic designs to support more critical movements.
REFERENCES: [1]Burma Children Medical Fund - Mae Sot, Thailand. BCMF | Burma Children Medical Fund - Mae Sot, Thailand - Operating to give people a future. (n.d.). https://burmachildren.com/ [2]Buchanan, J. (2018, March 27). Kwawu Arm 2.0 - Prosthetic - socket version. Thingiverse. https://www.thingiverse.com/thing:2841281 [3]NIOP. (2022, February 9). NIOP Q-C V1 quick-connect wrist. Thingiverse. http://www.thingiverse.com/thing:5238794 [4]OpenSCAD. The Programmers Solid 3D CAD Modeller. (n.d.). https://openscad.org/ [5]UN. Overview of Myanmar nationals in Thailand. IOM UN migration. https://thailand.iom.int/sites/g/files/tmzbdl1371/files/documents/2024-10/overview-of-myanmar-nationals-in-thailand-october-24.pdf
Elkind, Emese; Radcliffe, Olivia; Tun, Aung Tin; Connolly, Laura; Davison, Colleen; Purkey, Eva; Fichtinger, Gabor; Thornton, Kanchana
Strengthening Low-cost Prosthetic Solutions in Thailand/Myanmar Through Academic Institution-NGO Collaboration Honorable Mention Conference
Health & Human Rights Conference, Queen's University School of Medicine, 2025, (3rd place).
@conference{Elkind2025,
title = {Strengthening Low-cost Prosthetic Solutions in Thailand/Myanmar Through Academic Institution-NGO Collaboration },
author = {Emese Elkind and Olivia Radcliffe and Aung Tin Tun and Laura Connolly and Colleen Davison and Eva Purkey and Gabor Fichtinger and Kanchana Thornton
},
year = {2025},
date = {2025-02-22},
urldate = {2025-02-22},
booktitle = {Health & Human Rights Conference},
publisher = {School of Medicine},
organization = {Queen's University},
abstract = {The ongoing civil war in Myanmar, along with the related coup in 2021, have displaced millions of refugees to Thailand, where many lack immigration status and cannot access medical care. The Burma Children Medical Fund (BCMF) [1] addresses these challenges by providing funding and support for medical treatment, including a 3D-printed prosthetics program initiated in 2019 for individuals with limb loss. Due to limited Computer-Aided Design (CAD) experience, BCMF staff have turned to open-source prosthetic designs. We aim to establish an academia-NGO partnership to strengthen BCMF’s efforts, provide technical support, and broaden outreach to underserved communities needing low-cost, body-powered prosthetic devices. Our collaboration includes Queen’s University volunteers traveling to BCMF’s workshop for on-ground support and continuing remote assistance. As BCMF utilizes open-source prosthetic designs from platforms such as Thingiverse [2], we wanted to maintain the 3D printing workflow while addressing gaps in open-source prosthetic offerings. We identified three critical needs: devices for short-below-elbow amputees, above-elbow amputees, and a detachable, rotatable wrist. In response, we modified BCMF’s most used prosthetic design to customize the model for these specific needs. We conducted iterative testing for durability and comfort, ensuring constant communication between staff and recipients, allowing patient feedback to guide our designs. Over the past two years, Queen’s University has sent two volunteers to BCMF, with another planned for this year. So far, five recipients use our short-below-elbow prosthetic design, and one has received a quick connect wrist. In addition, we are currently collaborating remotely on a new prosthetic design for above-elbow amputees. This partnership between Queen’s University and BCMF improves access to low-cost prosthetic solutions, expands BCMF’s recipient pool, and demonstrates the potential for future partnerships between educational institutions and NGOs to address disparities in healthcare access.
References
[1] Burma Children Medical Fund - Mae Sot, Thailand. BCMF | Burma Children Medical Fund - Mae Sot, Thailand - Operating to give people a future. https://burmachildren.com/
[2] Buchanan, J. (2018, March 27). Kwawu Arm 2.0 - Prosthetic - socket version. Thingiverse. https://www.thingiverse.com/thing:2841281 },
note = {3rd place},
keywords = {},
pubstate = {published},
tppubtype = {conference}
}
References
[1] Burma Children Medical Fund - Mae Sot, Thailand. BCMF | Burma Children Medical Fund - Mae Sot, Thailand - Operating to give people a future. https://burmachildren.com/
[2] Buchanan, J. (2018, March 27). Kwawu Arm 2.0 - Prosthetic - socket version. Thingiverse. https://www.thingiverse.com/thing:2841281
Hashtrudi-Zaad, Kian; Farvolden, Coleman; Connolly, Laura; Barr, Colton; Fichtinger, Gabor
Robotic tracking of a resection cavity using a low cost bench-top robotic arm and electromagnetics Journal Article
In: vol. 13408, pp. 217-222, 2025.
@article{hashtrudi-zaad2025,
title = {Robotic tracking of a resection cavity using a low cost bench-top robotic arm and electromagnetics},
author = {Kian Hashtrudi-Zaad and Coleman Farvolden and Laura Connolly and Colton Barr and Gabor Fichtinger},
year = {2025},
date = {2025-01-01},
volume = {13408},
pages = {217-222},
publisher = {SPIE},
abstract = {INTRODUCTION
Roughly 40% of breast cancer patients are required to undergo corrective surgery after tumour resection via breast-conserving surgery (BCS). Sweeping of the cavity, resulting from the tumour resection, by spectroscopy and ultrasound imaging is emerging as a potential solution for identifying leftover cancer. However, the use of imaging modalities in the cavity is challenging as breast tissue is soft, malleable, and moves frequently. This paper presents and verifies an approach for tracking the relative motion of a resection cavity with a robotic arm.
METHODS
We use electromagnetic tracking and a low cost 6-axis robotic arm to track a simulated resection cavity. We embed an electromagnetic sensor in a 3D printed retractor that is designed to hold the cavity open. An open-source module in 3D Slicer is then used to detect cavity motion from the retractor and command the robotic arm to follow the …},
keywords = {},
pubstate = {published},
tppubtype = {article}
}
Roughly 40% of breast cancer patients are required to undergo corrective surgery after tumour resection via breast-conserving surgery (BCS). Sweeping of the cavity, resulting from the tumour resection, by spectroscopy and ultrasound imaging is emerging as a potential solution for identifying leftover cancer. However, the use of imaging modalities in the cavity is challenging as breast tissue is soft, malleable, and moves frequently. This paper presents and verifies an approach for tracking the relative motion of a resection cavity with a robotic arm.
METHODS
We use electromagnetic tracking and a low cost 6-axis robotic arm to track a simulated resection cavity. We embed an electromagnetic sensor in a 3D printed retractor that is designed to hold the cavity open. An open-source module in 3D Slicer is then used to detect cavity motion from the retractor and command the robotic arm to follow the …
Farvolden, Coleman; Hashtrudi-Zaad, Kian; Connolly, Laura; Barr, Colton; Fichtinger, Gabor
An accessible six-axis testbed for image-guided robotics research Journal Article
In: vol. 13408, pp. 458-463, 2025.
@article{farvolden2025,
title = {An accessible six-axis testbed for image-guided robotics research},
author = {Coleman Farvolden and Kian Hashtrudi-Zaad and Laura Connolly and Colton Barr and Gabor Fichtinger},
year = {2025},
date = {2025-01-01},
volume = {13408},
pages = {458-463},
publisher = {SPIE},
abstract = {PURPOSE: Cancer can recur after tumor resection surgery if tumor tissue is missed and left behind. We hypothesize that intraoperative robotic imaging could be used to inspect the surgical cavity and localize residual cancer tissue. This technique has the potential to improve the success rate of tumor resection surgery. Towards this, we propose and evaluate a benchtop testbed for robotic manipulation of an optical imaging probe. We use low-cost hardware and open-source software to construct the testbed and describe the implementation so that it can be easily adopted to support similar research. METHODS: We implemented a reusable, open-source module in 3D Slicer for reading position coordinates and motion planning with an inexpensive 6-axis robotic arm in Robot Operating System (ROS). For demonstration, a custom end-effector was used to fix an optical probe to the robot. The accuracy of the testbed …},
keywords = {},
pubstate = {published},
tppubtype = {article}
}
Elkind, Emese; Tun, Aung Tin; Radcliffe, Olivia; Connolly, Laura; Davison, Colleen; Purkey, Eva; Mousavi, Parvin; Fichtinger, Gabor; Thornton, Kanchana
Canadian Association for Global Health, 2024.
@conference{Elkind2024b,
title = {Enhancing healthcare access by developing low-cost 3D printed prosthetics along the Thai-Myanmar border},
author = {Emese Elkind and Aung Tin Tun and Olivia Radcliffe and Laura Connolly and Colleen Davison and Eva Purkey and Parvin Mousavi and Gabor Fichtinger and Kanchana Thornton
},
url = {https://labs.cs.queensu.ca/perklab/wp-content/uploads/sites/3/2024/10/EElkind_CCGH2024.pdf},
year = {2024},
date = {2024-10-25},
urldate = {2024-10-25},
publisher = {Canadian Association for Global Health},
abstract = {Background/Objective
Inadequacies in the Burmese healthcare system, heightened by the 2021 military coup of the civil war in Myanmar and the COVID-19 pandemic, have driven thousands of refugees to Thailand seeking medical aid. Without immigration status, these refugees, especially those who have experienced limb loss, are challenged by the inability to receive healthcare. Burma Children Medical Fund (BCMF, www.burmachildren.com) based in Mae Sot, Tak, Thailand focuses on funding underserved Burmese communities’ medical treatment and providing support services.
Prosthetics in lower-income countries are usually passive, therefore, patients cannot fully perform their daily functions, impacting their abilities to work and affecting family caretakers. BCMF aims to make body-powered prosthetics that work best in low-resource settings using open-source designs, which only allow for fixed hand positions. The usage of prosthetic arms depends heavily on their functionality and comfort. Patients are more likely to consistently use prosthetics if it aids them in returning to normalcy and reducing family burdens. My objective is to design an interchangeable hand to enable critical rotational movements.
Methodology
The BCMF prosthetics project makes custom-fitted, low-cost, 3D-printed prostheses. BCMF uses open-source prosthetic models such as the Kwawu Arm 2.0, which provides an OpenSCAD (openscad.org) file for adjusting the model to the recipient's measurements. To maintain BCMF’s workflow, the interchangeable wrist model was created using the 3D design software, Autodesk Fusion 360, and designs from NIOP Q-C v1 and v2 Quick-Connect Wrist. The wrist was merged onto the Kwawu Arm, printed, assembled, and tested. This is an iterative process where patient feedback ensures the prosthetics cater to the diverse needs of the recipients.
Results
Since the launch of the prosthetics project in 2019, BCMF has provided 3D-printed prosthetics to 76 patients. The interchangeable hand provides a solution to many patients' everyday activities and can rotate the hand 360 degrees.
Conclusions
This project provides a low-cost solution to healthcare challenges in the context of poly-crisis experienced in Myanmar, enhancing the resilience and adaptability of affected refugee communities.
Relevance to Sub-Theme
This presentation aligns with sub-theme 2 by developing and testing methods to improve healthcare access and quality in areas affected by war, migration, poverty, and racial disparities.},
keywords = {},
pubstate = {published},
tppubtype = {conference}
}
Inadequacies in the Burmese healthcare system, heightened by the 2021 military coup of the civil war in Myanmar and the COVID-19 pandemic, have driven thousands of refugees to Thailand seeking medical aid. Without immigration status, these refugees, especially those who have experienced limb loss, are challenged by the inability to receive healthcare. Burma Children Medical Fund (BCMF, www.burmachildren.com) based in Mae Sot, Tak, Thailand focuses on funding underserved Burmese communities’ medical treatment and providing support services.
Prosthetics in lower-income countries are usually passive, therefore, patients cannot fully perform their daily functions, impacting their abilities to work and affecting family caretakers. BCMF aims to make body-powered prosthetics that work best in low-resource settings using open-source designs, which only allow for fixed hand positions. The usage of prosthetic arms depends heavily on their functionality and comfort. Patients are more likely to consistently use prosthetics if it aids them in returning to normalcy and reducing family burdens. My objective is to design an interchangeable hand to enable critical rotational movements.
Methodology
The BCMF prosthetics project makes custom-fitted, low-cost, 3D-printed prostheses. BCMF uses open-source prosthetic models such as the Kwawu Arm 2.0, which provides an OpenSCAD (openscad.org) file for adjusting the model to the recipient's measurements. To maintain BCMF’s workflow, the interchangeable wrist model was created using the 3D design software, Autodesk Fusion 360, and designs from NIOP Q-C v1 and v2 Quick-Connect Wrist. The wrist was merged onto the Kwawu Arm, printed, assembled, and tested. This is an iterative process where patient feedback ensures the prosthetics cater to the diverse needs of the recipients.
Results
Since the launch of the prosthetics project in 2019, BCMF has provided 3D-printed prosthetics to 76 patients. The interchangeable hand provides a solution to many patients' everyday activities and can rotate the hand 360 degrees.
Conclusions
This project provides a low-cost solution to healthcare challenges in the context of poly-crisis experienced in Myanmar, enhancing the resilience and adaptability of affected refugee communities.
Relevance to Sub-Theme
This presentation aligns with sub-theme 2 by developing and testing methods to improve healthcare access and quality in areas affected by war, migration, poverty, and racial disparities.
Connolly, Laura; Kumar, Aravind S; Mehta, Kapi Ketan; Al-Zogbi, Lidia; Kazanzides, Peter; Mousavi, Parvin; Fichtinger, Gabor; Krieger, Axel; Tokuda, Junichi; Taylor, Russell H; Leonard, Simon; Deguet, Anton
SlicerROS2: A Research and Development Module for Image-Guided Robotic Interventions Journal Article
In: IEEE Transactions on Medical Robotics and Bionics, 2024.
@article{connolly2024,
title = {SlicerROS2: A Research and Development Module for Image-Guided Robotic Interventions},
author = {Laura Connolly and Aravind S Kumar and Kapi Ketan Mehta and Lidia Al-Zogbi and Peter Kazanzides and Parvin Mousavi and Gabor Fichtinger and Axel Krieger and Junichi Tokuda and Russell H Taylor and Simon Leonard and Anton Deguet},
year = {2024},
date = {2024-01-01},
journal = {IEEE Transactions on Medical Robotics and Bionics},
publisher = {IEEE},
keywords = {},
pubstate = {published},
tppubtype = {article}
}
Connolly, Laura; Fooladgar, Fahimeh; Jamzad, Amoon; Kaufmann, Martin; Syeda, Ayesha; Ren, Kevin; Abolmaesumi, Purang; Rudan, John F; McKay, Doug; Fichtinger, Gabor; Mousavi, Parvin
ImSpect: Image-driven self-supervised learning for surgical margin evaluation with mass spectrometry Journal Article
In: International Journal of Computer Assisted Radiology and Surgery, pp. 1-8, 2024.
@article{fichtinger2024e,
title = {ImSpect: Image-driven self-supervised learning for surgical margin evaluation with mass spectrometry},
author = {Laura Connolly and Fahimeh Fooladgar and Amoon Jamzad and Martin Kaufmann and Ayesha Syeda and Kevin Ren and Purang Abolmaesumi and John F Rudan and Doug McKay and Gabor Fichtinger and Parvin Mousavi},
url = {https://link.springer.com/article/10.1007/s11548-024-03106-1},
year = {2024},
date = {2024-01-01},
journal = {International Journal of Computer Assisted Radiology and Surgery},
pages = {1-8},
publisher = {Springer International Publishing},
abstract = {Purpose
Real-time assessment of surgical margins is critical for favorable outcomes in cancer patients. The iKnife is a mass spectrometry device that has demonstrated potential for margin detection in cancer surgery. Previous studies have shown that using deep learning on iKnife data can facilitate real-time tissue characterization. However, none of the existing literature on the iKnife facilitate the use of publicly available, state-of-the-art pretrained networks or datasets that have been used in computer vision and other domains.
Methods
In a new framework we call ImSpect, we convert 1D iKnife data, captured during basal cell carcinoma (BCC) surgery, into 2D images in order to capitalize on state-of-the-art image classification networks. We also use self-supervision to leverage large amounts of unlabeled, intraoperative data to accommodate the data requirements of these networks.
Results
Through extensive ablation …},
keywords = {},
pubstate = {published},
tppubtype = {article}
}
Real-time assessment of surgical margins is critical for favorable outcomes in cancer patients. The iKnife is a mass spectrometry device that has demonstrated potential for margin detection in cancer surgery. Previous studies have shown that using deep learning on iKnife data can facilitate real-time tissue characterization. However, none of the existing literature on the iKnife facilitate the use of publicly available, state-of-the-art pretrained networks or datasets that have been used in computer vision and other domains.
Methods
In a new framework we call ImSpect, we convert 1D iKnife data, captured during basal cell carcinoma (BCC) surgery, into 2D images in order to capitalize on state-of-the-art image classification networks. We also use self-supervision to leverage large amounts of unlabeled, intraoperative data to accommodate the data requirements of these networks.
Results
Through extensive ablation …
Radcliffe, Olivia; Connolly, Laura; Ungi, Tamas; Yeo, Caitlin; Rudan, John F.; Fichtinger, Gabor; Mousavi, Parvin
Navigated surgical resection cavity inspection for breast conserving surgery Proceedings
2023.
@proceedings{nokey,
title = {Navigated surgical resection cavity inspection for breast conserving surgery},
author = {Olivia Radcliffe and Laura Connolly and Tamas Ungi and Caitlin Yeo and John F. Rudan and Gabor Fichtinger and Parvin Mousavi},
doi = {https://doi.org/10.1117/12.2654015},
year = {2023},
date = {2023-04-03},
abstract = {Up to 40% of Breast Conserving Surgery (BCS) patients must undergo repeat surgery because cancer is left behind in the resection cavity. The mobility of the breast resection cavity makes it difficult to localize residual cancer and, therefore, cavity shaving is a common technique for cancer removal. Cavity shaving involves removing an additional layer of tissue from the entire resection cavity, often resulting in unnecessary healthy tissue loss. In this study, we demonstrated a navigation system and open-source software module that facilitates visualization of the breast resection cavity for targeted localization of residual cancer.},
keywords = {},
pubstate = {published},
tppubtype = {proceedings}
}
Morton, David; Connolly, Laura; Groves, Leah; Sunderland, Kyle; Jamzad, Amoon; Rudan, John F; Fichtinger, Gabor; Ungi, Tamas; Mousavi, Parvin
Tracked tissue sensing for tumor bed inspection Journal Article
In: vol. 12466, pp. 378-385, 2023.
@article{fichtinger2023x,
title = {Tracked tissue sensing for tumor bed inspection},
author = {David Morton and Laura Connolly and Leah Groves and Kyle Sunderland and Amoon Jamzad and John F Rudan and Gabor Fichtinger and Tamas Ungi and Parvin Mousavi},
url = {https://www.spiedigitallibrary.org/conference-proceedings-of-spie/12466/124661K/Tracked-tissue-sensing-for-tumor-bed-inspection/10.1117/12.2654217.short},
year = {2023},
date = {2023-01-01},
volume = {12466},
pages = {378-385},
publisher = {SPIE},
abstract = {Up to 30% of breast-conserving surgery patients require secondary surgery to remove cancerous tissue missed in the initial intervention. We hypothesize that tracked tissue sensing can improve the success rate of breast-conserving surgery. Tissue sensor tracking allows the surgeon to intraoperatively scan the tumor bed for leftover cancerous tissue. In this study, we characterize the performance of our tracked optical scanning testbed using an experimental pipeline. We assess the Dice similarity coefficient, accuracy, and latency of the testbed.},
keywords = {},
pubstate = {published},
tppubtype = {article}
}
Radcliffe, Olivia; Connolly, Laura; Ungi, Tamas; Yeo, Caitlin; Rudan, John F; Fichtinger, Gabor; Mousavi, Parvin
Navigated surgical resection cavity inspection for breast conserving surgery Journal Article
In: vol. 12466, pp. 234-241, 2023.
@article{fichtinger2023t,
title = {Navigated surgical resection cavity inspection for breast conserving surgery},
author = {Olivia Radcliffe and Laura Connolly and Tamas Ungi and Caitlin Yeo and John F Rudan and Gabor Fichtinger and Parvin Mousavi},
url = {https://www.spiedigitallibrary.org/conference-proceedings-of-spie/12466/124660Z/Navigated-surgical-resection-cavity-inspection-for-breast-conserving-surgery/10.1117/12.2654015.short},
year = {2023},
date = {2023-01-01},
volume = {12466},
pages = {234-241},
publisher = {SPIE},
abstract = {Up to 40% of Breast Conserving Surgery (BCS) patients must undergo repeat surgery because cancer is left behind in the resection cavity. The mobility of the breast resection cavity makes it difficult to localize residual cancer and, therefore, cavity shaving is a common technique for cancer removal. Cavity shaving involves removing an additional layer of tissue from the entire resection cavity, often resulting in unnecessary healthy tissue loss. In this study, we demonstrated a navigation system and open-source software module that facilitates visualization of the breast resection cavity for targeted localization of residual cancer.},
keywords = {},
pubstate = {published},
tppubtype = {article}
}
Jamzad, Amoon; Fooladgar, Fahimeh; Connolly, Laura; Srikanthan, Dilakshan; Syeda, Ayesha; Kaufmann, Martin; Ren, Kevin YM; Merchant, Shaila; Engel, Jay; Varma, Sonal; Fichtinger, Gabor; Rudan, John F; Mousavi, Parvin
Bridging Ex-Vivo Training and Intra-operative Deployment for Surgical Margin Assessment with Evidential Graph Transformer Journal Article
In: pp. 562-571, 2023.
@article{fichtinger2023g,
title = {Bridging Ex-Vivo Training and Intra-operative Deployment for Surgical Margin Assessment with Evidential Graph Transformer},
author = {Amoon Jamzad and Fahimeh Fooladgar and Laura Connolly and Dilakshan Srikanthan and Ayesha Syeda and Martin Kaufmann and Kevin YM Ren and Shaila Merchant and Jay Engel and Sonal Varma and Gabor Fichtinger and John F Rudan and Parvin Mousavi},
url = {https://link.springer.com/chapter/10.1007/978-3-031-43990-2_53},
year = {2023},
date = {2023-01-01},
pages = {562-571},
publisher = {Springer Nature Switzerland},
abstract = {PURPOSE
The use of intra-operative mass spectrometry along with Graph Transformer models showed promising results for margin detection on ex-vivo data. Although highly interpretable, these methods lack the ability to handle the uncertainty associated with intra-operative decision making. In this paper for the first time, we propose Evidential Graph Transformer network, a combination of attention mapping and uncertainty estimation to increase the performance and interpretability of surgical margin assessment.
METHODS
The Evidential Graph Transformer was formulated to output the uncertainty estimation along with intermediate attentions. The performance of the model was compared with different baselines in an ex-vivo cross-validation scheme, with extensive ablation study. The association of the model with clinical features were explored. The model was further validated for a prospective ex-vivo data, as …},
keywords = {},
pubstate = {published},
tppubtype = {article}
}
The use of intra-operative mass spectrometry along with Graph Transformer models showed promising results for margin detection on ex-vivo data. Although highly interpretable, these methods lack the ability to handle the uncertainty associated with intra-operative decision making. In this paper for the first time, we propose Evidential Graph Transformer network, a combination of attention mapping and uncertainty estimation to increase the performance and interpretability of surgical margin assessment.
METHODS
The Evidential Graph Transformer was formulated to output the uncertainty estimation along with intermediate attentions. The performance of the model was compared with different baselines in an ex-vivo cross-validation scheme, with extensive ablation study. The association of the model with clinical features were explored. The model was further validated for a prospective ex-vivo data, as …
Fooladgar, Fahimeh; Jamzad, Amoon; Connolly, Laura; Santilli, Alice; Kaufmann, Martin; Ren, Kevin; Abolmaesumi, Purang; Rudan, John; McKay, Doug; Fichtinger, Gabor; Mousavi, Parvin
Uncertainty estimation for margin detection in cancer surgery using mass spectrometry Journal Article
In: International Journal of Computer Assisted Radiology and Surgery, 2022.
@article{Fooladgar2022,
title = {Uncertainty estimation for margin detection in cancer surgery using mass spectrometry},
author = {Fahimeh Fooladgar and Amoon Jamzad and Laura Connolly and Alice Santilli and Martin Kaufmann and Kevin Ren and Purang Abolmaesumi and John Rudan and Doug McKay and Gabor Fichtinger and Parvin Mousavi},
doi = {https://doi.org/10.1007/s11548-022-02764-3},
year = {2022},
date = {2022-09-01},
journal = {International Journal of Computer Assisted Radiology and Surgery},
keywords = {},
pubstate = {published},
tppubtype = {article}
}
Connolly, Laura; Degeut, Anton; Leonard, Simon; Tokuda, Junichi; Ungi, Tamas; Krieger, Axel; Kazanzides, Peter; Mousavi, Parvin; Fichtinger, Gabor; Taylor, Russell H.
Bridging 3D Slicer and ROS2 for Image-Guided Robotic Interventions Journal Article
In: Sensors, vol. 22, 2022.
@article{Connolly2022c,
title = {Bridging 3D Slicer and ROS2 for Image-Guided Robotic Interventions},
author = {Laura Connolly and Anton Degeut and Simon Leonard and Junichi Tokuda and Tamas Ungi and Axel Krieger and Peter Kazanzides and Parvin Mousavi and Gabor Fichtinger and Russell H. Taylor},
doi = {https://doi.org/10.3390/s22145336},
year = {2022},
date = {2022-07-01},
journal = {Sensors},
volume = {22},
keywords = {},
pubstate = {published},
tppubtype = {article}
}
Connolly, Laura; Jamzad, Amoon; Nikniazi, Arash; Poushimin, Rana; Nunzi, Jean Michel; Rudan, John; Fichtinger, Gabor; Mousavi, Parvin
Feasibility of combined optical and acoustic imaging for surgical cavity scanning Conference
SPIE Medical Imaging 2022: Image-Guided Procedures, Robotic Interventions, and Modeling, vol. 12034, San Diego (online), 2022.
@conference{Connolly2022,
title = {Feasibility of combined optical and acoustic imaging for surgical cavity scanning},
author = {Laura Connolly and Amoon Jamzad and Arash Nikniazi and Rana Poushimin and Jean Michel Nunzi and John Rudan and Gabor Fichtinger and Parvin Mousavi},
doi = {https://doi.org/10.1117/12.2611964},
year = {2022},
date = {2022-04-01},
booktitle = {SPIE Medical Imaging 2022: Image-Guided Procedures, Robotic Interventions, and Modeling},
volume = {12034},
address = {San Diego (online)},
abstract = {PURPOSE: Over 30% of breast conserving surgery patients must undergo repeat surgery to address incomplete tumor resection. We hypothesize that the addition of a robotic cavity scanning system can improve the success rates of these procedures by performing additional, intraoperative imaging to detect left-over cancer cells. In this study, we assess the feasibility of a combined optical and acoustic imaging approach for this cavity scanning system. METHODS: Dual-layer tissue phantoms are imaged with both throughput broadband spectroscopy and an endocavity ultrasound probe. The absorbance and transmittance of the incident light from the broadband source is used to characterize each tissue sample optically. Additionally, a temporally enhanced ultrasound approach is used to distinguish the heterogeneity of the tissue sample by classifying individual pixels in the ultrasound image with a support vector machine. The goal of this combined approach is to use optical characterization to classify the tissue surface, and acoustic characterization to classify the sample heterogeneity. RESULTS: Both optical and acoustic characterization demonstrated promising preliminary results. The class of each tissue sample is distinctly separable based on the transmittance and absorption of the broadband light. Additionally, an SVM trained on the temporally enhance ultrasound signals for each tissue type, showed 82% linear separability of labelled temporally enhanced ultrasound sequences in our test set. CONCLUSIONS: By combining broadband and ultrasound imaging, we demonstrate a potential non-destructive imaging approach for this robotic cavity scanning system. With this approach, our system can detect both surface level tissue characteristics and depth information. Applying this to breast conserving surgery can help inform the surgeon about the tissue composition of the resection cavity after initial tumor resection.},
keywords = {},
pubstate = {published},
tppubtype = {conference}
}
Connolly, Laura; Deguet, Anton; Leonard, Simon; Tokuda, Junichi; Ungi, Tamas; Krieger, Axel; Kazanzides, Peter; Mousavi, Parvin; Fichtinger, Gabor; Taylor, Russell H
Bridging 3D Slicer and ROS2 for image-guided robotic interventions Journal Article
In: Sensors, vol. 22, iss. 14, pp. 5336, 2022.
@article{fichtinger2022e,
title = {Bridging 3D Slicer and ROS2 for image-guided robotic interventions},
author = {Laura Connolly and Anton Deguet and Simon Leonard and Junichi Tokuda and Tamas Ungi and Axel Krieger and Peter Kazanzides and Parvin Mousavi and Gabor Fichtinger and Russell H Taylor},
url = {https://www.mdpi.com/1424-8220/22/14/5336},
year = {2022},
date = {2022-01-01},
journal = {Sensors},
volume = {22},
issue = {14},
pages = {5336},
publisher = {MDPI},
abstract = {Developing image-guided robotic systems requires access to flexible, open-source software. For image guidance, the open-source medical imaging platform 3D Slicer is one of the most adopted tools that can be used for research and prototyping. Similarly, for robotics, the open-source middleware suite robot operating system (ROS) is the standard development framework. In the past, there have been several “ad hoc” attempts made to bridge both tools; however, they are all reliant on middleware and custom interfaces. Additionally, none of these attempts have been successful in bridging access to the full suite of tools provided by ROS or 3D Slicer. Therefore, in this paper, we present the SlicerROS2 module, which was designed for the direct use of ROS2 packages and libraries within 3D Slicer. The module was developed to enable real-time visualization of robots, accommodate different robot configurations, and facilitate data transfer in both directions (between ROS and Slicer). We demonstrate the system on multiple robots with different configurations, evaluate the system performance and discuss an image-guided robotic intervention that can be prototyped with this module. This module can serve as a starting point for clinical system development that reduces the need for custom interfaces and time-intensive platform setup.},
keywords = {},
pubstate = {published},
tppubtype = {article}
}
Connolly, Laura; Jamzad, Amoon; Nikniazi, Arash; Poushimin, Rana; Lasso, Andras; Sunderland, Kyle R.; Ungi, Tamas; Nunzi, Jean Michel; Rudan, John; Fichtinger, Gabor; Mousavi, Parvin
An open-source testbed for developing image-guided robotic tumor-bed inspection Conference
Imaging Network of Ontario (ImNO) Symposium, 2022.
@conference{connolly2022b,
title = {An open-source testbed for developing image-guided robotic tumor-bed inspection},
author = {Laura Connolly and Amoon Jamzad and Arash Nikniazi and Rana Poushimin and Andras Lasso and Kyle R. Sunderland and Tamas Ungi and Jean Michel Nunzi and John Rudan and Gabor Fichtinger and Parvin Mousavi},
url = {https://labs.cs.queensu.ca/perklab/wp-content/uploads/sites/3/2024/01/Connolly2022b.pdf},
year = {2022},
date = {2022-01-01},
urldate = {2022-01-01},
booktitle = {Imaging Network of Ontario (ImNO) Symposium},
keywords = {},
pubstate = {published},
tppubtype = {conference}
}
Connolly, Laura; Degeut, Anton; Sunderland, Kyle R.; Lasso, Andras; Ungi, Tamas; Rudan, John; Taylor, Russell H.; Mousavi, Parvin; Fichtinger, Gabor
An open-source platform for cooperative semi-autonomous robotic surgery Conference
IEEE International Conference on Autonomous Systems, IEEE IEEE, Montreal, Quebec, 2021.
@conference{Connolly2021,
title = {An open-source platform for cooperative semi-autonomous robotic surgery},
author = {Laura Connolly and Anton Degeut and Kyle R. Sunderland and Andras Lasso and Tamas Ungi and John Rudan and Russell H. Taylor and Parvin Mousavi and Gabor Fichtinger},
doi = {https://doi.org/10.1109/ICAS49788.2021.9551149},
year = {2021},
date = {2021-10-01},
urldate = {2021-10-01},
booktitle = {IEEE International Conference on Autonomous Systems},
publisher = {IEEE},
address = {Montreal, Quebec},
organization = {IEEE},
keywords = {},
pubstate = {published},
tppubtype = {conference}
}
Connolly, Laura; Jamzad, Amoon; Kaufmann, Martin; Farquharson, Catriona E.; Ren, Kevin; Rudan, John; Fichtinger, Gabor; Mousavi, Parvin
Combined Mass Spectrometry and Histopathology Imaging for Perioperative Tissue Assessment in Cancer Surgery Journal Article
In: Journal of Imaging, vol. 7, no. 203, 2021.
@article{Connolly2021c,
title = {Combined Mass Spectrometry and Histopathology Imaging for Perioperative Tissue Assessment in Cancer Surgery},
author = {Laura Connolly and Amoon Jamzad and Martin Kaufmann and Catriona E. Farquharson and Kevin Ren and John Rudan and Gabor Fichtinger and Parvin Mousavi},
doi = {https://doi.org/10.3390/jimaging7100203},
year = {2021},
date = {2021-10-01},
journal = {Journal of Imaging},
volume = {7},
number = {203},
abstract = {<p><span style="color:rgb(34, 34, 34); font-family:arial; font-size:12px">Mass spectrometry is an effective imaging tool for evaluating biological tissue to detect cancer. With the assistance of deep learning, this technology can be used as a perioperative tissue assessment tool that will facilitate informed surgical decisions. To achieve such a system requires the development of a database of mass spectrometry signals and their corresponding pathology labels. Assigning correct labels, in turn, necessitates precise spatial registration of histopathology and mass spectrometry data. This is a challenging task due to the domain differences and noisy nature of images. In this study, we create a registration framework for mass spectrometry and pathology images as a contribution to the development of perioperative tissue assessment. In doing so, we explore two opportunities in deep learning for medical image registration, namely, unsupervised, multi-modal deformable image registration and evaluation of the registration. We test this system on prostate needle biopsy cores that were imaged with desorption electrospray ionization mass spectrometry (DESI) and show that we can successfully register DESI and histology images to achieve accurate alignment and, consequently, labelling for future training. This automation is expected to improve the efficiency and development of a deep learning architecture that will benefit the use of mass spectrometry imaging for cancer diagnosis.</span></p>},
keywords = {},
pubstate = {published},
tppubtype = {article}
}
Connolly, Laura; Jamzad, Amoon; Kaufmann, Martin; Farquharson, Catriona E; Ren, Kevin; Rudan, John F; Fichtinger, Gabor; Mousavi, Parvin
Combined mass spectrometry and histopathology imaging for perioperative tissue assessment in cancer surgery Journal Article
In: Journal of Imaging, vol. 7, iss. 10, pp. 203, 2021.
@article{fichtinger2021f,
title = {Combined mass spectrometry and histopathology imaging for perioperative tissue assessment in cancer surgery},
author = {Laura Connolly and Amoon Jamzad and Martin Kaufmann and Catriona E Farquharson and Kevin Ren and John F Rudan and Gabor Fichtinger and Parvin Mousavi},
url = {https://www.mdpi.com/2313-433X/7/10/203},
year = {2021},
date = {2021-01-01},
journal = {Journal of Imaging},
volume = {7},
issue = {10},
pages = {203},
publisher = {MDPI},
abstract = {Mass spectrometry is an effective imaging tool for evaluating biological tissue to detect cancer. With the assistance of deep learning, this technology can be used as a perioperative tissue assessment tool that will facilitate informed surgical decisions. To achieve such a system requires the development of a database of mass spectrometry signals and their corresponding pathology labels. Assigning correct labels, in turn, necessitates precise spatial registration of histopathology and mass spectrometry data. This is a challenging task due to the domain differences and noisy nature of images. In this study, we create a registration framework for mass spectrometry and pathology images as a contribution to the development of perioperative tissue assessment. In doing so, we explore two opportunities in deep learning for medical image registration, namely, unsupervised, multi-modal deformable image registration and evaluation of the registration. We test this system on prostate needle biopsy cores that were imaged with desorption electrospray ionization mass spectrometry (DESI) and show that we can successfully register DESI and histology images to achieve accurate alignment and, consequently, labelling for future training. This automation is expected to improve the efficiency and development of a deep learning architecture that will benefit the use of mass spectrometry imaging for cancer diagnosis.},
keywords = {},
pubstate = {published},
tppubtype = {article}
}
Akbarifar, Faranak; Jamzad, Amoon; Santilli, Alice; Kauffman, Martin; Janssen, Natasja; Connolly, Laura; Ren, K; Vanderbeck, Kaitlin; Wang, Ami; Mckay, Doug; Rudan, John; Fichtinger, Gabor; Mousavi, Parvin
Graph-based analysis of mass spectrometry data for tissue characterization with application in basal cell carcinoma surgery Journal Article
In: vol. 11598, pp. 279-285, 2021.
@article{fichtinger2021e,
title = {Graph-based analysis of mass spectrometry data for tissue characterization with application in basal cell carcinoma surgery},
author = {Faranak Akbarifar and Amoon Jamzad and Alice Santilli and Martin Kauffman and Natasja Janssen and Laura Connolly and K Ren and Kaitlin Vanderbeck and Ami Wang and Doug Mckay and John Rudan and Gabor Fichtinger and Parvin Mousavi},
url = {https://www.spiedigitallibrary.org/conference-proceedings-of-spie/11598/1159812/Graph-based-analysis-of-mass-spectrometry-data-for-tissue-characterization/10.1117/12.2582045.short},
year = {2021},
date = {2021-01-01},
volume = {11598},
pages = {279-285},
publisher = {SPIE},
abstract = {PURPOSE
Basal Cell Carcinoma (BCC) is the most common cancer in the world. Surgery is the standard treatment and margin assessment is used to evaluate the outcome. The presence of cancerous cells at the edge of resected tissue i.e., positive margin, can negatively impact patient outcomes and increase the probability of cancer recurrence. Novel mass spectrometry technologies paired with machine learning can provide surgeons with real-time feedback about margins to eliminate the need for resurgery. To our knowledge, this is the first study to report the performance of cancer detection using Graph Convolutional Networks (GCN) on mass spectrometry data from resected BCC samples.
METHODS
The dataset used in this study is a subset of an ongoing clinical data acquired by our group and annotated with the help of a trained pathologist. There is a total number of 190 spectra in this dataset, including 127 …},
keywords = {},
pubstate = {published},
tppubtype = {article}
}
Basal Cell Carcinoma (BCC) is the most common cancer in the world. Surgery is the standard treatment and margin assessment is used to evaluate the outcome. The presence of cancerous cells at the edge of resected tissue i.e., positive margin, can negatively impact patient outcomes and increase the probability of cancer recurrence. Novel mass spectrometry technologies paired with machine learning can provide surgeons with real-time feedback about margins to eliminate the need for resurgery. To our knowledge, this is the first study to report the performance of cancer detection using Graph Convolutional Networks (GCN) on mass spectrometry data from resected BCC samples.
METHODS
The dataset used in this study is a subset of an ongoing clinical data acquired by our group and annotated with the help of a trained pathologist. There is a total number of 190 spectra in this dataset, including 127 …
Connolly, Laura; Deguet, Anton; Sunderland, Kyle; Lasso, Andras; Ungi, Tamas; Rudan, John F; Taylor, Russell H; Mousavi, Parvin; Fichtinger, Gabor
An open-source platform for cooperative, semi-autonomous robotic surgery Journal Article
In: pp. 1-5, 2021.
@article{fichtinger2021d,
title = {An open-source platform for cooperative, semi-autonomous robotic surgery},
author = {Laura Connolly and Anton Deguet and Kyle Sunderland and Andras Lasso and Tamas Ungi and John F Rudan and Russell H Taylor and Parvin Mousavi and Gabor Fichtinger},
url = {https://ieeexplore.ieee.org/abstract/document/9551149/},
year = {2021},
date = {2021-01-01},
pages = {1-5},
publisher = {IEEE},
abstract = {Introduction
In this paper, we present and assess a proof of concept platform for semi-autonomous, cooperative robotic surgery. The platform is easily reproducible thanks to simple hardware components and open-source software. Moreover, the design accommodates open, soft tissue surgeries that recent advancements in surgical robotics do not generally focus on.
Methods
The system is made up of an inexpensive robotic manipulator, a navigation system and a software interface. Accuracy measurement is performed on a rigid phantom that mimics the conditions of breast conserving surgery (BCS) as an example of a surgical use case.
Results
The average target registration error (TRE) and fiducial registration error (FRE) of the system is within 1 mm. This indicates that the navigation system is sufficient for certain surgical applications such as BCS. The platform can also be easily replicated and used in a lab or …},
keywords = {},
pubstate = {published},
tppubtype = {article}
}
In this paper, we present and assess a proof of concept platform for semi-autonomous, cooperative robotic surgery. The platform is easily reproducible thanks to simple hardware components and open-source software. Moreover, the design accommodates open, soft tissue surgeries that recent advancements in surgical robotics do not generally focus on.
Methods
The system is made up of an inexpensive robotic manipulator, a navigation system and a software interface. Accuracy measurement is performed on a rigid phantom that mimics the conditions of breast conserving surgery (BCS) as an example of a surgical use case.
Results
The average target registration error (TRE) and fiducial registration error (FRE) of the system is within 1 mm. This indicates that the navigation system is sufficient for certain surgical applications such as BCS. The platform can also be easily replicated and used in a lab or …
Connolly, Laura; Sunderland, Kyle R.; Lasso, Andras; Degeut, Anton; Ungi, Tamas; Rudan, John; Taylor, Russell H.; Mousavi, Parvin; Fichtinger, Gabor
A platform for robot-assisted Intraoperative imaging in breast conserving surgery Conference
Imaging Network of Ontario Symposium, Imaging Network of Ontario Symposium, Online, 2021.
@conference{Connolly2021b,
title = {A platform for robot-assisted Intraoperative imaging in breast conserving surgery},
author = {Laura Connolly and Kyle R. Sunderland and Andras Lasso and Anton Degeut and Tamas Ungi and John Rudan and Russell H. Taylor and Parvin Mousavi and Gabor Fichtinger},
url = {https://labs.cs.queensu.ca/perklab/wp-content/uploads/sites/3/2024/02/Connolly2021a_1.pdf},
year = {2021},
date = {2021-01-01},
urldate = {2021-01-01},
booktitle = {Imaging Network of Ontario Symposium},
publisher = {Imaging Network of Ontario Symposium},
address = {Online},
keywords = {},
pubstate = {published},
tppubtype = {conference}
}
Yates, Lauren; Connolly, Laura; Jamzad, Amoon; Asselin, Mark; Rubino, Rachel; Yam, Scott; Ungi, Tamas; Lasso, Andras; Nicol, Christopher; Mousavi, Parvin; Fichtinger, Gabor
Robotic tissue scanning with biophotonic probe Journal Article
In: vol. 11315, pp. 330-335, 2020.
@article{fichtinger2020o,
title = {Robotic tissue scanning with biophotonic probe},
author = {Lauren Yates and Laura Connolly and Amoon Jamzad and Mark Asselin and Rachel Rubino and Scott Yam and Tamas Ungi and Andras Lasso and Christopher Nicol and Parvin Mousavi and Gabor Fichtinger},
url = {https://www.spiedigitallibrary.org/conference-proceedings-of-spie/11315/1131519/Robotic-tissue-scanning-with-biophotonic-probe/10.1117/12.2549635.short},
year = {2020},
date = {2020-01-01},
volume = {11315},
pages = {330-335},
publisher = {SPIE},
abstract = {PURPOSE
Raman spectroscopy is an optical imaging technique used to characterize tissue via molecular analysis. The use of Raman spectroscopy for real-time intraoperative tissue classification requires fast analysis with minimal human intervention. In order to have accurate predictions and classifications, a large and reliable database of tissue classifications with spectra results is required. We have developed a system that can be used to generate an efficient scanning path for robotic scanning of tissues using Raman spectroscopy.
METHODS
A camera mounted to a robotic controller is used to take an image of a tissue slide. The corners of the tissue slides within the sample image are identified, and the size of the slide is calculated. The image is cropped to fit the size of the slide and the image is manipulated to identify the tissue contour. A grid set to fit around the size of the tissue is calculated and a grid …},
keywords = {},
pubstate = {published},
tppubtype = {article}
}
Raman spectroscopy is an optical imaging technique used to characterize tissue via molecular analysis. The use of Raman spectroscopy for real-time intraoperative tissue classification requires fast analysis with minimal human intervention. In order to have accurate predictions and classifications, a large and reliable database of tissue classifications with spectra results is required. We have developed a system that can be used to generate an efficient scanning path for robotic scanning of tissues using Raman spectroscopy.
METHODS
A camera mounted to a robotic controller is used to take an image of a tissue slide. The corners of the tissue slides within the sample image are identified, and the size of the slide is calculated. The image is cropped to fit the size of the slide and the image is manipulated to identify the tissue contour. A grid set to fit around the size of the tissue is calculated and a grid …
Santilli, Alice ML; Jamzad, Amoon; Janssen, Natasja NY; Kaufmann, Martin; Connolly, Laura; Vanderbeck, Kaitlin; Wang, Ami; McKay, Doug; Rudan, John F; Fichtinger, Gabor; Mousavi, Parvin
Perioperative margin detection in basal cell carcinoma using a deep learning framework: a feasibility study Journal Article
In: International Journal of Computer Assisted Radiology and Surgery, vol. 15, pp. 887-896, 2020.
@article{fichtinger2020b,
title = {Perioperative margin detection in basal cell carcinoma using a deep learning framework: a feasibility study},
author = {Alice ML Santilli and Amoon Jamzad and Natasja NY Janssen and Martin Kaufmann and Laura Connolly and Kaitlin Vanderbeck and Ami Wang and Doug McKay and John F Rudan and Gabor Fichtinger and Parvin Mousavi},
url = {https://link.springer.com/article/10.1007/s11548-020-02152-9},
year = {2020},
date = {2020-01-01},
journal = {International Journal of Computer Assisted Radiology and Surgery},
volume = {15},
pages = {887-896},
publisher = {Springer International Publishing},
abstract = {Purpose
Basal cell carcinoma (BCC) is the most commonly diagnosed cancer and the number of diagnosis is growing worldwide due to increased exposure to solar radiation and the aging population. Reduction of positive margin rates when removing BCC leads to fewer revision surgeries and consequently lower health care costs, improved cosmetic outcomes and better patient care. In this study, we propose the first use of a perioperative mass spectrometry technology (iKnife) along with a deep learning framework for detection of BCC signatures from tissue burns.
Methods
Resected surgical specimen were collected and inspected by a pathologist. With their guidance, data were collected by burning regions of the specimen labeled as BCC or normal, with the iKnife. Data included 190 scans of which 127 were normal and 63 were BCC. A data …},
keywords = {},
pubstate = {published},
tppubtype = {article}
}
Basal cell carcinoma (BCC) is the most commonly diagnosed cancer and the number of diagnosis is growing worldwide due to increased exposure to solar radiation and the aging population. Reduction of positive margin rates when removing BCC leads to fewer revision surgeries and consequently lower health care costs, improved cosmetic outcomes and better patient care. In this study, we propose the first use of a perioperative mass spectrometry technology (iKnife) along with a deep learning framework for detection of BCC signatures from tissue burns.
Methods
Resected surgical specimen were collected and inspected by a pathologist. With their guidance, data were collected by burning regions of the specimen labeled as BCC or normal, with the iKnife. Data included 190 scans of which 127 were normal and 63 were BCC. A data …
Connolly, Laura; Jamzad, Amoon; Kaufmann, Martin; Rubino, Rachel; Sedghi, Alireza; Ungi, Tamas; Asselin, Mark; Yam, Scott; Rudan, John; Nicol, Christopher; Fichtinger, Gabor; Mousavi, Parvin
Medical Imaging 2020: Image-Guided Procedures, Robotic Interventions and Modeling, vol. 11315, SPIE SPIE, Houston, Texas, United States, 2020.
@conference{Connolly2020a,
title = {Classification of tumor signatures from electrosurgical vapors using mass spectrometry and machine learning: a feasibility study},
author = {Laura Connolly and Amoon Jamzad and Martin Kaufmann and Rachel Rubino and Alireza Sedghi and Tamas Ungi and Mark Asselin and Scott Yam and John Rudan and Christopher Nicol and Gabor Fichtinger and Parvin Mousavi},
url = {https://labs.cs.queensu.ca/perklab/wp-content/uploads/sites/3/2024/02/Connolly2020a.pdf},
doi = {https://doi.org/10.1117/12.2549343},
year = {2020},
date = {2020-01-01},
urldate = {2020-01-01},
booktitle = {Medical Imaging 2020: Image-Guided Procedures, Robotic Interventions and Modeling},
volume = {11315},
publisher = {SPIE},
address = {Houston, Texas, United States},
organization = {SPIE},
keywords = {},
pubstate = {published},
tppubtype = {conference}
}
Connolly, Laura; Ungi, Tamas; Lasso, Andras; Vaughan, Thomas; Asselin, Mark; Mousavi, Parvin; Yam, Scott; Fichtinger, Gabor
Mechanically-Controlled Spectroscopic Imaging for Tissue Classification Conference
SPIE Medical Imaging 2019: Image-Guided Procedures, Robotic Interventions, and Modeling, vol. 10951, San Diego, California, 2019.
@conference{Connolly2019a,
title = {Mechanically-Controlled Spectroscopic Imaging for Tissue Classification},
author = {Laura Connolly and Tamas Ungi and Andras Lasso and Thomas Vaughan and Mark Asselin and Parvin Mousavi and Scott Yam and Gabor Fichtinger},
url = {https://labs.cs.queensu.ca/perklab/wp-content/uploads/sites/3/2024/02/Connolly2019a_3.pdf},
doi = {https://doi.org/10.1117/12.2512481},
year = {2019},
date = {2019-03-01},
urldate = {2019-03-01},
booktitle = {SPIE Medical Imaging 2019: Image-Guided Procedures, Robotic Interventions, and Modeling},
volume = {10951},
address = {San Diego, California},
keywords = {},
pubstate = {published},
tppubtype = {conference}
}
Connolly, Laura; Ungi, Tamas; Lasso, Andras; Vaughan, Thomas; Asselin, Mark; Mousavi, Parvin; Yam, Scott; Fichtinger, Gabor
Mechanically controlled spectroscopic imaging for tissue classification Journal Article
In: vol. 10951, pp. 632-640, 2019.
@article{fichtinger2019n,
title = {Mechanically controlled spectroscopic imaging for tissue classification},
author = {Laura Connolly and Tamas Ungi and Andras Lasso and Thomas Vaughan and Mark Asselin and Parvin Mousavi and Scott Yam and Gabor Fichtinger},
url = {https://www.spiedigitallibrary.org/conference-proceedings-of-spie/10951/109512E/Mechanically-controlled-spectroscopic-imaging-for-tissue-classification/10.1117/12.2512481.short},
year = {2019},
date = {2019-01-01},
volume = {10951},
pages = {632-640},
publisher = {SPIE},
abstract = {PURPOSE
Raman Spectroscopy is amongst several optical imaging techniques that have the ability to characterize tissue non-invasively. To use these technologies for intraoperative tissue classification, fast and efficient analysis of optical data is required with minimal operator intervention. Additionally, there is a need for a reliable database of optical signatures to account for variable conditions. We developed a software system with an inexpensive, flexible mechanical framework to facilitate automated scanning of tissue and validate spectroscopic scans with histologic ground truths. This system will be used, in the future, to train a machine learning algorithm to distinguish between different tissue types using Raman Spectroscopy.
METHODS
A sample of chicken breast tissue is mounted to a microscope slide following a biopsy of fresh frozen tissue. Landmarks for registration and evaluation are marked on the …},
keywords = {},
pubstate = {published},
tppubtype = {article}
}
Raman Spectroscopy is amongst several optical imaging techniques that have the ability to characterize tissue non-invasively. To use these technologies for intraoperative tissue classification, fast and efficient analysis of optical data is required with minimal operator intervention. Additionally, there is a need for a reliable database of optical signatures to account for variable conditions. We developed a software system with an inexpensive, flexible mechanical framework to facilitate automated scanning of tissue and validate spectroscopic scans with histologic ground truths. This system will be used, in the future, to train a machine learning algorithm to distinguish between different tissue types using Raman Spectroscopy.
METHODS
A sample of chicken breast tissue is mounted to a microscope slide following a biopsy of fresh frozen tissue. Landmarks for registration and evaluation are marked on the …